Do I really need to solve 5 nonlinear equations in this Lagrange multiplier problem?
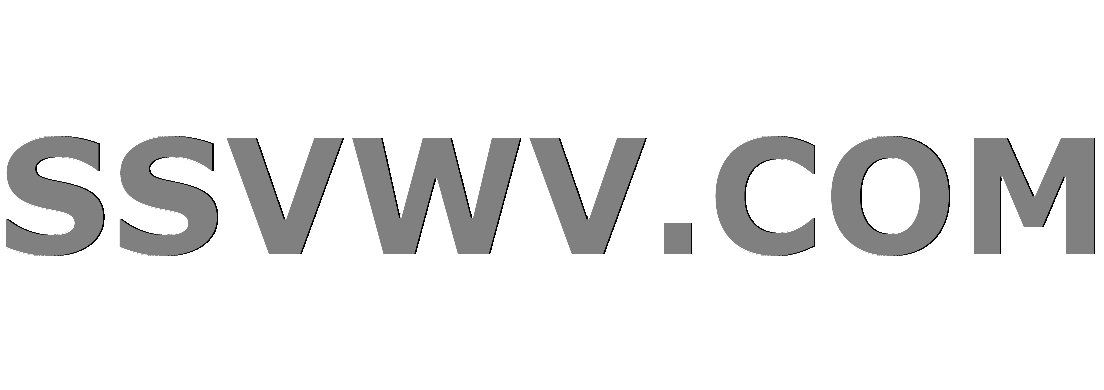
Multi tool use
$begingroup$
I need to solve the following optimization problem
Let $X=left{ x_{i}right} _{i=1}^{n}$ be an independent sequence of $k$-face die rolls. Where for $jinleft[kright]$ we have $pleft(x_{i}=jright)=theta_{j}$ (So $sum_{i=1}^{k}theta_{i}=1$)
I am requested to find the ML estimator for $theta$ for $k=3$ under the constraint $theta_{1}=theta_{2}+theta_{3}$
My work so far:
The log likelihood function is given by $$ellleft(thetamid Xright)=log p_{theta}left(Xright)=logprod_{i=1}^{n}p_{theta}left(x_{i}right)=sum_{i=1}^{n}log p_{theta}left(x_{i}right)=sum_{i=1}^{n}logtheta_{x_{i}}=sum_{i=1}^{k}n_{i}logtheta_{i}$$
where $n_{i}$ is the number of occurrences of face $i$
So the appropriate Lagrangian function is
$$mathcal{L}left(thetaright)=ellleft(thetamid Xright)-lambda_{0}left(sum_{i=1}^{k}theta_{i}-1right)-lambda_{1}left(theta_{1}-theta_{2}-theta_{3}right)=$$
$$sum_{i=1}^{3}n_{i}logtheta_{i}-lambda_{0}left(theta_{1}+theta_{2}+theta_{3}-1right)-lambda_{1}left(theta_{1}-theta_{2}-theta_{3}right)$$
and then we need to solve
$$nablamathcal{L}=left(begin{matrix}frac{partialmathcal{L}}{partialtheta_{1}}\
frac{partialmathcal{L}}{partialtheta_{2}}\
frac{partialmathcal{L}}{partialtheta_{3}}\
frac{partialmathcal{L}}{partiallambda_{0}}\
frac{partialmathcal{L}}{partiallambda_{1}}
end{matrix}right)=left(begin{matrix}frac{n_{1}}{theta_{1}}-lambda_{0}-lambda_{1}\
frac{n_{2}}{theta_{2}}-lambda_{0}+lambda_{1}\
frac{n_{3}}{theta_{3}}-lambda_{0}+lambda_{1}\
-theta_{1}-theta_{2}-theta_{3}+1\
-theta_{1}+theta_{2}+theta_{3}
end{matrix}right)=left(begin{matrix}0\
0\
0\
0\
0
end{matrix}right)$$
Is this analysis correct? If so, do I really have to solve 5 (non linear) equations with 5 unknowns to get the answer?
This is my first time working with Lagrange multipliers, and it seems to me that either I am wrong here or else there is some kind of a workaround, especially since the following questions seem like they'l end up with even more equations.
systems-of-equations lagrange-multiplier maximum-likelihood
$endgroup$
add a comment |
$begingroup$
I need to solve the following optimization problem
Let $X=left{ x_{i}right} _{i=1}^{n}$ be an independent sequence of $k$-face die rolls. Where for $jinleft[kright]$ we have $pleft(x_{i}=jright)=theta_{j}$ (So $sum_{i=1}^{k}theta_{i}=1$)
I am requested to find the ML estimator for $theta$ for $k=3$ under the constraint $theta_{1}=theta_{2}+theta_{3}$
My work so far:
The log likelihood function is given by $$ellleft(thetamid Xright)=log p_{theta}left(Xright)=logprod_{i=1}^{n}p_{theta}left(x_{i}right)=sum_{i=1}^{n}log p_{theta}left(x_{i}right)=sum_{i=1}^{n}logtheta_{x_{i}}=sum_{i=1}^{k}n_{i}logtheta_{i}$$
where $n_{i}$ is the number of occurrences of face $i$
So the appropriate Lagrangian function is
$$mathcal{L}left(thetaright)=ellleft(thetamid Xright)-lambda_{0}left(sum_{i=1}^{k}theta_{i}-1right)-lambda_{1}left(theta_{1}-theta_{2}-theta_{3}right)=$$
$$sum_{i=1}^{3}n_{i}logtheta_{i}-lambda_{0}left(theta_{1}+theta_{2}+theta_{3}-1right)-lambda_{1}left(theta_{1}-theta_{2}-theta_{3}right)$$
and then we need to solve
$$nablamathcal{L}=left(begin{matrix}frac{partialmathcal{L}}{partialtheta_{1}}\
frac{partialmathcal{L}}{partialtheta_{2}}\
frac{partialmathcal{L}}{partialtheta_{3}}\
frac{partialmathcal{L}}{partiallambda_{0}}\
frac{partialmathcal{L}}{partiallambda_{1}}
end{matrix}right)=left(begin{matrix}frac{n_{1}}{theta_{1}}-lambda_{0}-lambda_{1}\
frac{n_{2}}{theta_{2}}-lambda_{0}+lambda_{1}\
frac{n_{3}}{theta_{3}}-lambda_{0}+lambda_{1}\
-theta_{1}-theta_{2}-theta_{3}+1\
-theta_{1}+theta_{2}+theta_{3}
end{matrix}right)=left(begin{matrix}0\
0\
0\
0\
0
end{matrix}right)$$
Is this analysis correct? If so, do I really have to solve 5 (non linear) equations with 5 unknowns to get the answer?
This is my first time working with Lagrange multipliers, and it seems to me that either I am wrong here or else there is some kind of a workaround, especially since the following questions seem like they'l end up with even more equations.
systems-of-equations lagrange-multiplier maximum-likelihood
$endgroup$
add a comment |
$begingroup$
I need to solve the following optimization problem
Let $X=left{ x_{i}right} _{i=1}^{n}$ be an independent sequence of $k$-face die rolls. Where for $jinleft[kright]$ we have $pleft(x_{i}=jright)=theta_{j}$ (So $sum_{i=1}^{k}theta_{i}=1$)
I am requested to find the ML estimator for $theta$ for $k=3$ under the constraint $theta_{1}=theta_{2}+theta_{3}$
My work so far:
The log likelihood function is given by $$ellleft(thetamid Xright)=log p_{theta}left(Xright)=logprod_{i=1}^{n}p_{theta}left(x_{i}right)=sum_{i=1}^{n}log p_{theta}left(x_{i}right)=sum_{i=1}^{n}logtheta_{x_{i}}=sum_{i=1}^{k}n_{i}logtheta_{i}$$
where $n_{i}$ is the number of occurrences of face $i$
So the appropriate Lagrangian function is
$$mathcal{L}left(thetaright)=ellleft(thetamid Xright)-lambda_{0}left(sum_{i=1}^{k}theta_{i}-1right)-lambda_{1}left(theta_{1}-theta_{2}-theta_{3}right)=$$
$$sum_{i=1}^{3}n_{i}logtheta_{i}-lambda_{0}left(theta_{1}+theta_{2}+theta_{3}-1right)-lambda_{1}left(theta_{1}-theta_{2}-theta_{3}right)$$
and then we need to solve
$$nablamathcal{L}=left(begin{matrix}frac{partialmathcal{L}}{partialtheta_{1}}\
frac{partialmathcal{L}}{partialtheta_{2}}\
frac{partialmathcal{L}}{partialtheta_{3}}\
frac{partialmathcal{L}}{partiallambda_{0}}\
frac{partialmathcal{L}}{partiallambda_{1}}
end{matrix}right)=left(begin{matrix}frac{n_{1}}{theta_{1}}-lambda_{0}-lambda_{1}\
frac{n_{2}}{theta_{2}}-lambda_{0}+lambda_{1}\
frac{n_{3}}{theta_{3}}-lambda_{0}+lambda_{1}\
-theta_{1}-theta_{2}-theta_{3}+1\
-theta_{1}+theta_{2}+theta_{3}
end{matrix}right)=left(begin{matrix}0\
0\
0\
0\
0
end{matrix}right)$$
Is this analysis correct? If so, do I really have to solve 5 (non linear) equations with 5 unknowns to get the answer?
This is my first time working with Lagrange multipliers, and it seems to me that either I am wrong here or else there is some kind of a workaround, especially since the following questions seem like they'l end up with even more equations.
systems-of-equations lagrange-multiplier maximum-likelihood
$endgroup$
I need to solve the following optimization problem
Let $X=left{ x_{i}right} _{i=1}^{n}$ be an independent sequence of $k$-face die rolls. Where for $jinleft[kright]$ we have $pleft(x_{i}=jright)=theta_{j}$ (So $sum_{i=1}^{k}theta_{i}=1$)
I am requested to find the ML estimator for $theta$ for $k=3$ under the constraint $theta_{1}=theta_{2}+theta_{3}$
My work so far:
The log likelihood function is given by $$ellleft(thetamid Xright)=log p_{theta}left(Xright)=logprod_{i=1}^{n}p_{theta}left(x_{i}right)=sum_{i=1}^{n}log p_{theta}left(x_{i}right)=sum_{i=1}^{n}logtheta_{x_{i}}=sum_{i=1}^{k}n_{i}logtheta_{i}$$
where $n_{i}$ is the number of occurrences of face $i$
So the appropriate Lagrangian function is
$$mathcal{L}left(thetaright)=ellleft(thetamid Xright)-lambda_{0}left(sum_{i=1}^{k}theta_{i}-1right)-lambda_{1}left(theta_{1}-theta_{2}-theta_{3}right)=$$
$$sum_{i=1}^{3}n_{i}logtheta_{i}-lambda_{0}left(theta_{1}+theta_{2}+theta_{3}-1right)-lambda_{1}left(theta_{1}-theta_{2}-theta_{3}right)$$
and then we need to solve
$$nablamathcal{L}=left(begin{matrix}frac{partialmathcal{L}}{partialtheta_{1}}\
frac{partialmathcal{L}}{partialtheta_{2}}\
frac{partialmathcal{L}}{partialtheta_{3}}\
frac{partialmathcal{L}}{partiallambda_{0}}\
frac{partialmathcal{L}}{partiallambda_{1}}
end{matrix}right)=left(begin{matrix}frac{n_{1}}{theta_{1}}-lambda_{0}-lambda_{1}\
frac{n_{2}}{theta_{2}}-lambda_{0}+lambda_{1}\
frac{n_{3}}{theta_{3}}-lambda_{0}+lambda_{1}\
-theta_{1}-theta_{2}-theta_{3}+1\
-theta_{1}+theta_{2}+theta_{3}
end{matrix}right)=left(begin{matrix}0\
0\
0\
0\
0
end{matrix}right)$$
Is this analysis correct? If so, do I really have to solve 5 (non linear) equations with 5 unknowns to get the answer?
This is my first time working with Lagrange multipliers, and it seems to me that either I am wrong here or else there is some kind of a workaround, especially since the following questions seem like they'l end up with even more equations.
systems-of-equations lagrange-multiplier maximum-likelihood
systems-of-equations lagrange-multiplier maximum-likelihood
asked Dec 11 '18 at 20:59
D.M. D.M.
494
494
add a comment |
add a comment |
1 Answer
1
active
oldest
votes
$begingroup$
These aren't that hard to solve, despite being nonlinear. Referring to the equations by row number, $0=(2)-(3)$ gives $theta_3 = n_3theta_2/n_2$.
Next, $0=(4)-(5)=-2theta_2+1-2theta_3$. Plug in your $theta_3$ to solve for $theta_2$. Keep going...
$endgroup$
add a comment |
Your Answer
StackExchange.ifUsing("editor", function () {
return StackExchange.using("mathjaxEditing", function () {
StackExchange.MarkdownEditor.creationCallbacks.add(function (editor, postfix) {
StackExchange.mathjaxEditing.prepareWmdForMathJax(editor, postfix, [["$", "$"], ["\\(","\\)"]]);
});
});
}, "mathjax-editing");
StackExchange.ready(function() {
var channelOptions = {
tags: "".split(" "),
id: "69"
};
initTagRenderer("".split(" "), "".split(" "), channelOptions);
StackExchange.using("externalEditor", function() {
// Have to fire editor after snippets, if snippets enabled
if (StackExchange.settings.snippets.snippetsEnabled) {
StackExchange.using("snippets", function() {
createEditor();
});
}
else {
createEditor();
}
});
function createEditor() {
StackExchange.prepareEditor({
heartbeatType: 'answer',
autoActivateHeartbeat: false,
convertImagesToLinks: true,
noModals: true,
showLowRepImageUploadWarning: true,
reputationToPostImages: 10,
bindNavPrevention: true,
postfix: "",
imageUploader: {
brandingHtml: "Powered by u003ca class="icon-imgur-white" href="https://imgur.com/"u003eu003c/au003e",
contentPolicyHtml: "User contributions licensed under u003ca href="https://creativecommons.org/licenses/by-sa/3.0/"u003ecc by-sa 3.0 with attribution requiredu003c/au003e u003ca href="https://stackoverflow.com/legal/content-policy"u003e(content policy)u003c/au003e",
allowUrls: true
},
noCode: true, onDemand: true,
discardSelector: ".discard-answer"
,immediatelyShowMarkdownHelp:true
});
}
});
Sign up or log in
StackExchange.ready(function () {
StackExchange.helpers.onClickDraftSave('#login-link');
});
Sign up using Google
Sign up using Facebook
Sign up using Email and Password
Post as a guest
Required, but never shown
StackExchange.ready(
function () {
StackExchange.openid.initPostLogin('.new-post-login', 'https%3a%2f%2fmath.stackexchange.com%2fquestions%2f3035813%2fdo-i-really-need-to-solve-5-nonlinear-equations-in-this-lagrange-multiplier-prob%23new-answer', 'question_page');
}
);
Post as a guest
Required, but never shown
1 Answer
1
active
oldest
votes
1 Answer
1
active
oldest
votes
active
oldest
votes
active
oldest
votes
$begingroup$
These aren't that hard to solve, despite being nonlinear. Referring to the equations by row number, $0=(2)-(3)$ gives $theta_3 = n_3theta_2/n_2$.
Next, $0=(4)-(5)=-2theta_2+1-2theta_3$. Plug in your $theta_3$ to solve for $theta_2$. Keep going...
$endgroup$
add a comment |
$begingroup$
These aren't that hard to solve, despite being nonlinear. Referring to the equations by row number, $0=(2)-(3)$ gives $theta_3 = n_3theta_2/n_2$.
Next, $0=(4)-(5)=-2theta_2+1-2theta_3$. Plug in your $theta_3$ to solve for $theta_2$. Keep going...
$endgroup$
add a comment |
$begingroup$
These aren't that hard to solve, despite being nonlinear. Referring to the equations by row number, $0=(2)-(3)$ gives $theta_3 = n_3theta_2/n_2$.
Next, $0=(4)-(5)=-2theta_2+1-2theta_3$. Plug in your $theta_3$ to solve for $theta_2$. Keep going...
$endgroup$
These aren't that hard to solve, despite being nonlinear. Referring to the equations by row number, $0=(2)-(3)$ gives $theta_3 = n_3theta_2/n_2$.
Next, $0=(4)-(5)=-2theta_2+1-2theta_3$. Plug in your $theta_3$ to solve for $theta_2$. Keep going...
answered Dec 11 '18 at 21:18
Alex R.Alex R.
24.9k12452
24.9k12452
add a comment |
add a comment |
Thanks for contributing an answer to Mathematics Stack Exchange!
- Please be sure to answer the question. Provide details and share your research!
But avoid …
- Asking for help, clarification, or responding to other answers.
- Making statements based on opinion; back them up with references or personal experience.
Use MathJax to format equations. MathJax reference.
To learn more, see our tips on writing great answers.
Sign up or log in
StackExchange.ready(function () {
StackExchange.helpers.onClickDraftSave('#login-link');
});
Sign up using Google
Sign up using Facebook
Sign up using Email and Password
Post as a guest
Required, but never shown
StackExchange.ready(
function () {
StackExchange.openid.initPostLogin('.new-post-login', 'https%3a%2f%2fmath.stackexchange.com%2fquestions%2f3035813%2fdo-i-really-need-to-solve-5-nonlinear-equations-in-this-lagrange-multiplier-prob%23new-answer', 'question_page');
}
);
Post as a guest
Required, but never shown
Sign up or log in
StackExchange.ready(function () {
StackExchange.helpers.onClickDraftSave('#login-link');
});
Sign up using Google
Sign up using Facebook
Sign up using Email and Password
Post as a guest
Required, but never shown
Sign up or log in
StackExchange.ready(function () {
StackExchange.helpers.onClickDraftSave('#login-link');
});
Sign up using Google
Sign up using Facebook
Sign up using Email and Password
Post as a guest
Required, but never shown
Sign up or log in
StackExchange.ready(function () {
StackExchange.helpers.onClickDraftSave('#login-link');
});
Sign up using Google
Sign up using Facebook
Sign up using Email and Password
Sign up using Google
Sign up using Facebook
Sign up using Email and Password
Post as a guest
Required, but never shown
Required, but never shown
Required, but never shown
Required, but never shown
Required, but never shown
Required, but never shown
Required, but never shown
Required, but never shown
Required, but never shown
ZJ,vyRtr6eDOQQ0u6twEH929xHA0gG5eU,xL