How to convert constrainted optimization problem to an unconstrained one?
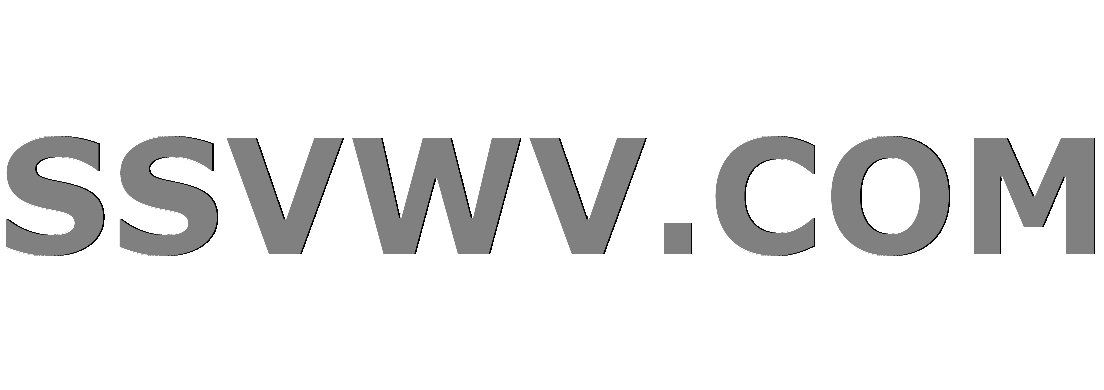
Multi tool use
$begingroup$
Suppose I have an optimization problem
$$ mathbf{w}^* = max_{mathbf{w}} sum_{i=1}^{N} (y_i - mathbf{w}cdotmathbf{x}_i)^2 $$
$$text{subject to}$$
$$sum_{j=1}^m w_j^2 leq tau$$
I want to show this can be written as
$$ mathbf{w}^* = max_{mathbf{w}} sum_{i=1}^{N} (y_i - mathbf{w}cdotmathbf{x}_i)^2 + lambda sum_{j=1}^m w_j^2$$
What is the basic intuition behind this?
I have done basic Lagrangian multiplier problems like
$$max_{mathbf{x}} U(mathbf{x})$$
$$text{subject to}$$
$$mathbf{x}cdot mathbf{w} = tau$$
In those cases, I have written a Lagrangian like
$$L(mathbf{x},lambda) = U(mathbf{x}) + lambda (m - mathbf{x}cdot mathbf{w})$$
But there's two issues:
- A Lagrangian isn't itself something I minimize, correct? I am trying to rewrite my constrained problem as an unconstrained problem.
- There is an inequality, not an equality. I assume the Kuhn-Tucker conditions are relevant to this, but I am not sure how.
optimization lagrange-multiplier
$endgroup$
add a comment |
$begingroup$
Suppose I have an optimization problem
$$ mathbf{w}^* = max_{mathbf{w}} sum_{i=1}^{N} (y_i - mathbf{w}cdotmathbf{x}_i)^2 $$
$$text{subject to}$$
$$sum_{j=1}^m w_j^2 leq tau$$
I want to show this can be written as
$$ mathbf{w}^* = max_{mathbf{w}} sum_{i=1}^{N} (y_i - mathbf{w}cdotmathbf{x}_i)^2 + lambda sum_{j=1}^m w_j^2$$
What is the basic intuition behind this?
I have done basic Lagrangian multiplier problems like
$$max_{mathbf{x}} U(mathbf{x})$$
$$text{subject to}$$
$$mathbf{x}cdot mathbf{w} = tau$$
In those cases, I have written a Lagrangian like
$$L(mathbf{x},lambda) = U(mathbf{x}) + lambda (m - mathbf{x}cdot mathbf{w})$$
But there's two issues:
- A Lagrangian isn't itself something I minimize, correct? I am trying to rewrite my constrained problem as an unconstrained problem.
- There is an inequality, not an equality. I assume the Kuhn-Tucker conditions are relevant to this, but I am not sure how.
optimization lagrange-multiplier
$endgroup$
$begingroup$
if an optimal solution to your original problem exists, what you want to show follows trivially from Lagrange duality (although determining $lambda$ is not trivial).
$endgroup$
– LinAlg
Oct 19 '18 at 0:02
$begingroup$
@LinAlg What information could I add to this post that would make it more answerable but that also wouldn't be like posting the original problem?
$endgroup$
– Stan Shunpike
Oct 19 '18 at 1:31
$begingroup$
I'm afraid I do not know what you are looking for. Lagrange duality means $min_x max_lambda L(x,lambda) = max_lambda min_x L(x,lambda)$, and you are rewriting the formulation on the left hand side to the one on the right hand side.
$endgroup$
– LinAlg
Oct 19 '18 at 1:57
$begingroup$
I've updated the question with a related one that will hopefully be more detailed.
$endgroup$
– Stan Shunpike
Oct 19 '18 at 6:32
add a comment |
$begingroup$
Suppose I have an optimization problem
$$ mathbf{w}^* = max_{mathbf{w}} sum_{i=1}^{N} (y_i - mathbf{w}cdotmathbf{x}_i)^2 $$
$$text{subject to}$$
$$sum_{j=1}^m w_j^2 leq tau$$
I want to show this can be written as
$$ mathbf{w}^* = max_{mathbf{w}} sum_{i=1}^{N} (y_i - mathbf{w}cdotmathbf{x}_i)^2 + lambda sum_{j=1}^m w_j^2$$
What is the basic intuition behind this?
I have done basic Lagrangian multiplier problems like
$$max_{mathbf{x}} U(mathbf{x})$$
$$text{subject to}$$
$$mathbf{x}cdot mathbf{w} = tau$$
In those cases, I have written a Lagrangian like
$$L(mathbf{x},lambda) = U(mathbf{x}) + lambda (m - mathbf{x}cdot mathbf{w})$$
But there's two issues:
- A Lagrangian isn't itself something I minimize, correct? I am trying to rewrite my constrained problem as an unconstrained problem.
- There is an inequality, not an equality. I assume the Kuhn-Tucker conditions are relevant to this, but I am not sure how.
optimization lagrange-multiplier
$endgroup$
Suppose I have an optimization problem
$$ mathbf{w}^* = max_{mathbf{w}} sum_{i=1}^{N} (y_i - mathbf{w}cdotmathbf{x}_i)^2 $$
$$text{subject to}$$
$$sum_{j=1}^m w_j^2 leq tau$$
I want to show this can be written as
$$ mathbf{w}^* = max_{mathbf{w}} sum_{i=1}^{N} (y_i - mathbf{w}cdotmathbf{x}_i)^2 + lambda sum_{j=1}^m w_j^2$$
What is the basic intuition behind this?
I have done basic Lagrangian multiplier problems like
$$max_{mathbf{x}} U(mathbf{x})$$
$$text{subject to}$$
$$mathbf{x}cdot mathbf{w} = tau$$
In those cases, I have written a Lagrangian like
$$L(mathbf{x},lambda) = U(mathbf{x}) + lambda (m - mathbf{x}cdot mathbf{w})$$
But there's two issues:
- A Lagrangian isn't itself something I minimize, correct? I am trying to rewrite my constrained problem as an unconstrained problem.
- There is an inequality, not an equality. I assume the Kuhn-Tucker conditions are relevant to this, but I am not sure how.
optimization lagrange-multiplier
optimization lagrange-multiplier
edited Oct 19 '18 at 6:31
Stan Shunpike
asked Oct 18 '18 at 21:49
Stan ShunpikeStan Shunpike
1,81111438
1,81111438
$begingroup$
if an optimal solution to your original problem exists, what you want to show follows trivially from Lagrange duality (although determining $lambda$ is not trivial).
$endgroup$
– LinAlg
Oct 19 '18 at 0:02
$begingroup$
@LinAlg What information could I add to this post that would make it more answerable but that also wouldn't be like posting the original problem?
$endgroup$
– Stan Shunpike
Oct 19 '18 at 1:31
$begingroup$
I'm afraid I do not know what you are looking for. Lagrange duality means $min_x max_lambda L(x,lambda) = max_lambda min_x L(x,lambda)$, and you are rewriting the formulation on the left hand side to the one on the right hand side.
$endgroup$
– LinAlg
Oct 19 '18 at 1:57
$begingroup$
I've updated the question with a related one that will hopefully be more detailed.
$endgroup$
– Stan Shunpike
Oct 19 '18 at 6:32
add a comment |
$begingroup$
if an optimal solution to your original problem exists, what you want to show follows trivially from Lagrange duality (although determining $lambda$ is not trivial).
$endgroup$
– LinAlg
Oct 19 '18 at 0:02
$begingroup$
@LinAlg What information could I add to this post that would make it more answerable but that also wouldn't be like posting the original problem?
$endgroup$
– Stan Shunpike
Oct 19 '18 at 1:31
$begingroup$
I'm afraid I do not know what you are looking for. Lagrange duality means $min_x max_lambda L(x,lambda) = max_lambda min_x L(x,lambda)$, and you are rewriting the formulation on the left hand side to the one on the right hand side.
$endgroup$
– LinAlg
Oct 19 '18 at 1:57
$begingroup$
I've updated the question with a related one that will hopefully be more detailed.
$endgroup$
– Stan Shunpike
Oct 19 '18 at 6:32
$begingroup$
if an optimal solution to your original problem exists, what you want to show follows trivially from Lagrange duality (although determining $lambda$ is not trivial).
$endgroup$
– LinAlg
Oct 19 '18 at 0:02
$begingroup$
if an optimal solution to your original problem exists, what you want to show follows trivially from Lagrange duality (although determining $lambda$ is not trivial).
$endgroup$
– LinAlg
Oct 19 '18 at 0:02
$begingroup$
@LinAlg What information could I add to this post that would make it more answerable but that also wouldn't be like posting the original problem?
$endgroup$
– Stan Shunpike
Oct 19 '18 at 1:31
$begingroup$
@LinAlg What information could I add to this post that would make it more answerable but that also wouldn't be like posting the original problem?
$endgroup$
– Stan Shunpike
Oct 19 '18 at 1:31
$begingroup$
I'm afraid I do not know what you are looking for. Lagrange duality means $min_x max_lambda L(x,lambda) = max_lambda min_x L(x,lambda)$, and you are rewriting the formulation on the left hand side to the one on the right hand side.
$endgroup$
– LinAlg
Oct 19 '18 at 1:57
$begingroup$
I'm afraid I do not know what you are looking for. Lagrange duality means $min_x max_lambda L(x,lambda) = max_lambda min_x L(x,lambda)$, and you are rewriting the formulation on the left hand side to the one on the right hand side.
$endgroup$
– LinAlg
Oct 19 '18 at 1:57
$begingroup$
I've updated the question with a related one that will hopefully be more detailed.
$endgroup$
– Stan Shunpike
Oct 19 '18 at 6:32
$begingroup$
I've updated the question with a related one that will hopefully be more detailed.
$endgroup$
– Stan Shunpike
Oct 19 '18 at 6:32
add a comment |
1 Answer
1
active
oldest
votes
$begingroup$
Introducing the slack variable $epsilon$ you can write the unconstrained equivalent optimization problem with the Lagrangian
$$
L(mathbf{w},lambda,epsilon) = sum_{i=1}^{N} (y_i - mathbf{w}cdotmathbf{x}_i)^2 + lambda left(sum_{j=1}^m w_j^2-tau + epsilon^2right)
$$
NOTES
1) Solving the lagrangian we will have it's stationary points which should be qualified.
2) Analyzing the the values for $epsilon^*, lambda^*$ we can conclude the K-T conditions.
$endgroup$
add a comment |
Your Answer
StackExchange.ifUsing("editor", function () {
return StackExchange.using("mathjaxEditing", function () {
StackExchange.MarkdownEditor.creationCallbacks.add(function (editor, postfix) {
StackExchange.mathjaxEditing.prepareWmdForMathJax(editor, postfix, [["$", "$"], ["\\(","\\)"]]);
});
});
}, "mathjax-editing");
StackExchange.ready(function() {
var channelOptions = {
tags: "".split(" "),
id: "69"
};
initTagRenderer("".split(" "), "".split(" "), channelOptions);
StackExchange.using("externalEditor", function() {
// Have to fire editor after snippets, if snippets enabled
if (StackExchange.settings.snippets.snippetsEnabled) {
StackExchange.using("snippets", function() {
createEditor();
});
}
else {
createEditor();
}
});
function createEditor() {
StackExchange.prepareEditor({
heartbeatType: 'answer',
autoActivateHeartbeat: false,
convertImagesToLinks: true,
noModals: true,
showLowRepImageUploadWarning: true,
reputationToPostImages: 10,
bindNavPrevention: true,
postfix: "",
imageUploader: {
brandingHtml: "Powered by u003ca class="icon-imgur-white" href="https://imgur.com/"u003eu003c/au003e",
contentPolicyHtml: "User contributions licensed under u003ca href="https://creativecommons.org/licenses/by-sa/3.0/"u003ecc by-sa 3.0 with attribution requiredu003c/au003e u003ca href="https://stackoverflow.com/legal/content-policy"u003e(content policy)u003c/au003e",
allowUrls: true
},
noCode: true, onDemand: true,
discardSelector: ".discard-answer"
,immediatelyShowMarkdownHelp:true
});
}
});
Sign up or log in
StackExchange.ready(function () {
StackExchange.helpers.onClickDraftSave('#login-link');
});
Sign up using Google
Sign up using Facebook
Sign up using Email and Password
Post as a guest
Required, but never shown
StackExchange.ready(
function () {
StackExchange.openid.initPostLogin('.new-post-login', 'https%3a%2f%2fmath.stackexchange.com%2fquestions%2f2961279%2fhow-to-convert-constrainted-optimization-problem-to-an-unconstrained-one%23new-answer', 'question_page');
}
);
Post as a guest
Required, but never shown
1 Answer
1
active
oldest
votes
1 Answer
1
active
oldest
votes
active
oldest
votes
active
oldest
votes
$begingroup$
Introducing the slack variable $epsilon$ you can write the unconstrained equivalent optimization problem with the Lagrangian
$$
L(mathbf{w},lambda,epsilon) = sum_{i=1}^{N} (y_i - mathbf{w}cdotmathbf{x}_i)^2 + lambda left(sum_{j=1}^m w_j^2-tau + epsilon^2right)
$$
NOTES
1) Solving the lagrangian we will have it's stationary points which should be qualified.
2) Analyzing the the values for $epsilon^*, lambda^*$ we can conclude the K-T conditions.
$endgroup$
add a comment |
$begingroup$
Introducing the slack variable $epsilon$ you can write the unconstrained equivalent optimization problem with the Lagrangian
$$
L(mathbf{w},lambda,epsilon) = sum_{i=1}^{N} (y_i - mathbf{w}cdotmathbf{x}_i)^2 + lambda left(sum_{j=1}^m w_j^2-tau + epsilon^2right)
$$
NOTES
1) Solving the lagrangian we will have it's stationary points which should be qualified.
2) Analyzing the the values for $epsilon^*, lambda^*$ we can conclude the K-T conditions.
$endgroup$
add a comment |
$begingroup$
Introducing the slack variable $epsilon$ you can write the unconstrained equivalent optimization problem with the Lagrangian
$$
L(mathbf{w},lambda,epsilon) = sum_{i=1}^{N} (y_i - mathbf{w}cdotmathbf{x}_i)^2 + lambda left(sum_{j=1}^m w_j^2-tau + epsilon^2right)
$$
NOTES
1) Solving the lagrangian we will have it's stationary points which should be qualified.
2) Analyzing the the values for $epsilon^*, lambda^*$ we can conclude the K-T conditions.
$endgroup$
Introducing the slack variable $epsilon$ you can write the unconstrained equivalent optimization problem with the Lagrangian
$$
L(mathbf{w},lambda,epsilon) = sum_{i=1}^{N} (y_i - mathbf{w}cdotmathbf{x}_i)^2 + lambda left(sum_{j=1}^m w_j^2-tau + epsilon^2right)
$$
NOTES
1) Solving the lagrangian we will have it's stationary points which should be qualified.
2) Analyzing the the values for $epsilon^*, lambda^*$ we can conclude the K-T conditions.
answered Dec 5 '18 at 17:04
CesareoCesareo
8,6393516
8,6393516
add a comment |
add a comment |
Thanks for contributing an answer to Mathematics Stack Exchange!
- Please be sure to answer the question. Provide details and share your research!
But avoid …
- Asking for help, clarification, or responding to other answers.
- Making statements based on opinion; back them up with references or personal experience.
Use MathJax to format equations. MathJax reference.
To learn more, see our tips on writing great answers.
Sign up or log in
StackExchange.ready(function () {
StackExchange.helpers.onClickDraftSave('#login-link');
});
Sign up using Google
Sign up using Facebook
Sign up using Email and Password
Post as a guest
Required, but never shown
StackExchange.ready(
function () {
StackExchange.openid.initPostLogin('.new-post-login', 'https%3a%2f%2fmath.stackexchange.com%2fquestions%2f2961279%2fhow-to-convert-constrainted-optimization-problem-to-an-unconstrained-one%23new-answer', 'question_page');
}
);
Post as a guest
Required, but never shown
Sign up or log in
StackExchange.ready(function () {
StackExchange.helpers.onClickDraftSave('#login-link');
});
Sign up using Google
Sign up using Facebook
Sign up using Email and Password
Post as a guest
Required, but never shown
Sign up or log in
StackExchange.ready(function () {
StackExchange.helpers.onClickDraftSave('#login-link');
});
Sign up using Google
Sign up using Facebook
Sign up using Email and Password
Post as a guest
Required, but never shown
Sign up or log in
StackExchange.ready(function () {
StackExchange.helpers.onClickDraftSave('#login-link');
});
Sign up using Google
Sign up using Facebook
Sign up using Email and Password
Sign up using Google
Sign up using Facebook
Sign up using Email and Password
Post as a guest
Required, but never shown
Required, but never shown
Required, but never shown
Required, but never shown
Required, but never shown
Required, but never shown
Required, but never shown
Required, but never shown
Required, but never shown
hj2mTi,zYqzl0 8iRJKiyafQf,ciBjSQgsAECcTWOc,YA3 oL0sR tdnOn
$begingroup$
if an optimal solution to your original problem exists, what you want to show follows trivially from Lagrange duality (although determining $lambda$ is not trivial).
$endgroup$
– LinAlg
Oct 19 '18 at 0:02
$begingroup$
@LinAlg What information could I add to this post that would make it more answerable but that also wouldn't be like posting the original problem?
$endgroup$
– Stan Shunpike
Oct 19 '18 at 1:31
$begingroup$
I'm afraid I do not know what you are looking for. Lagrange duality means $min_x max_lambda L(x,lambda) = max_lambda min_x L(x,lambda)$, and you are rewriting the formulation on the left hand side to the one on the right hand side.
$endgroup$
– LinAlg
Oct 19 '18 at 1:57
$begingroup$
I've updated the question with a related one that will hopefully be more detailed.
$endgroup$
– Stan Shunpike
Oct 19 '18 at 6:32