Total Least Square fitting
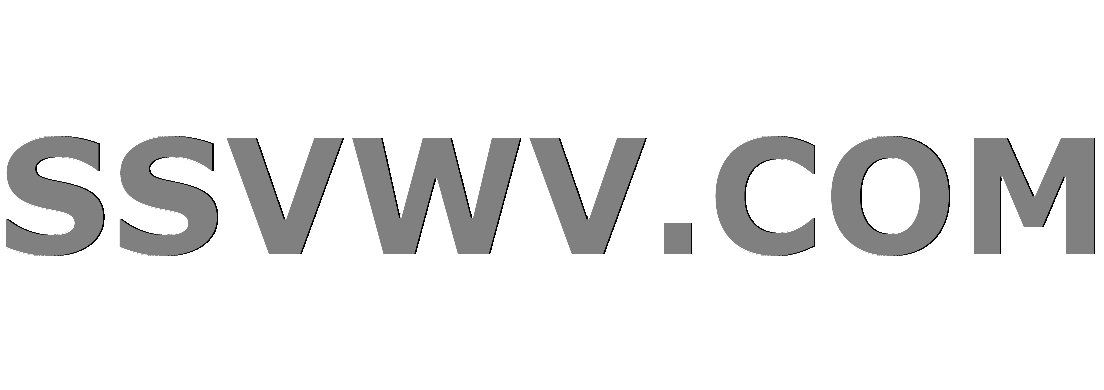
Multi tool use
$begingroup$
Say I want to fit a straight line using Total Least Square (as opposed to Least Square), which is to minimize the sum of (yi-k*xi-b)^2/(k^2+1) over all xi's and yi's, where xi's and yi's are training data point coordinates, and k and b are the fitting parameters.
I use gradient descent to solve for k and b. The cost function is just the above sum. But the result turns out that the cost isn't monotonic, it goes down and goes up. It's not supposed to be so, as this sum has 1 global minimum only. I don't know what goes wrong to make the cost function non monotonic?
ordinary-differential-equations least-squares linear-regression gradient-descent
$endgroup$
add a comment |
$begingroup$
Say I want to fit a straight line using Total Least Square (as opposed to Least Square), which is to minimize the sum of (yi-k*xi-b)^2/(k^2+1) over all xi's and yi's, where xi's and yi's are training data point coordinates, and k and b are the fitting parameters.
I use gradient descent to solve for k and b. The cost function is just the above sum. But the result turns out that the cost isn't monotonic, it goes down and goes up. It's not supposed to be so, as this sum has 1 global minimum only. I don't know what goes wrong to make the cost function non monotonic?
ordinary-differential-equations least-squares linear-regression gradient-descent
$endgroup$
$begingroup$
How could we see what goes wrong in your calculus without without having your calculus in detail ?
$endgroup$
– JJacquelin
Dec 10 '18 at 14:25
$begingroup$
Even for a simple quadratic function, gradient descent isn't guaranteed to converge if you don't pick a stepsize small enough. Consider for instance $f(x)=x^2$. If your stepsize is $h=1$, then $x_{n+1}=x_n-hf'(x_n)=x_n-2x_n=-x_n$ simply oscillates and never reaches the unique minimum.
$endgroup$
– Federico
Dec 10 '18 at 14:31
$begingroup$
@ JJacquelin I guarantee the rest of my calculus and gradient descent is correct. May you confirm that the above sum is correct?
$endgroup$
– feynman
Dec 13 '18 at 7:00
$begingroup$
@ Federico I guarantee my gradient descent calculation is correct, and step lengths are nothing to worry about because I use matlab ode23 package to solve. I didn't set the step lengths.
$endgroup$
– feynman
Dec 13 '18 at 7:01
add a comment |
$begingroup$
Say I want to fit a straight line using Total Least Square (as opposed to Least Square), which is to minimize the sum of (yi-k*xi-b)^2/(k^2+1) over all xi's and yi's, where xi's and yi's are training data point coordinates, and k and b are the fitting parameters.
I use gradient descent to solve for k and b. The cost function is just the above sum. But the result turns out that the cost isn't monotonic, it goes down and goes up. It's not supposed to be so, as this sum has 1 global minimum only. I don't know what goes wrong to make the cost function non monotonic?
ordinary-differential-equations least-squares linear-regression gradient-descent
$endgroup$
Say I want to fit a straight line using Total Least Square (as opposed to Least Square), which is to minimize the sum of (yi-k*xi-b)^2/(k^2+1) over all xi's and yi's, where xi's and yi's are training data point coordinates, and k and b are the fitting parameters.
I use gradient descent to solve for k and b. The cost function is just the above sum. But the result turns out that the cost isn't monotonic, it goes down and goes up. It's not supposed to be so, as this sum has 1 global minimum only. I don't know what goes wrong to make the cost function non monotonic?
ordinary-differential-equations least-squares linear-regression gradient-descent
ordinary-differential-equations least-squares linear-regression gradient-descent
asked Dec 10 '18 at 14:10
feynmanfeynman
1061
1061
$begingroup$
How could we see what goes wrong in your calculus without without having your calculus in detail ?
$endgroup$
– JJacquelin
Dec 10 '18 at 14:25
$begingroup$
Even for a simple quadratic function, gradient descent isn't guaranteed to converge if you don't pick a stepsize small enough. Consider for instance $f(x)=x^2$. If your stepsize is $h=1$, then $x_{n+1}=x_n-hf'(x_n)=x_n-2x_n=-x_n$ simply oscillates and never reaches the unique minimum.
$endgroup$
– Federico
Dec 10 '18 at 14:31
$begingroup$
@ JJacquelin I guarantee the rest of my calculus and gradient descent is correct. May you confirm that the above sum is correct?
$endgroup$
– feynman
Dec 13 '18 at 7:00
$begingroup$
@ Federico I guarantee my gradient descent calculation is correct, and step lengths are nothing to worry about because I use matlab ode23 package to solve. I didn't set the step lengths.
$endgroup$
– feynman
Dec 13 '18 at 7:01
add a comment |
$begingroup$
How could we see what goes wrong in your calculus without without having your calculus in detail ?
$endgroup$
– JJacquelin
Dec 10 '18 at 14:25
$begingroup$
Even for a simple quadratic function, gradient descent isn't guaranteed to converge if you don't pick a stepsize small enough. Consider for instance $f(x)=x^2$. If your stepsize is $h=1$, then $x_{n+1}=x_n-hf'(x_n)=x_n-2x_n=-x_n$ simply oscillates and never reaches the unique minimum.
$endgroup$
– Federico
Dec 10 '18 at 14:31
$begingroup$
@ JJacquelin I guarantee the rest of my calculus and gradient descent is correct. May you confirm that the above sum is correct?
$endgroup$
– feynman
Dec 13 '18 at 7:00
$begingroup$
@ Federico I guarantee my gradient descent calculation is correct, and step lengths are nothing to worry about because I use matlab ode23 package to solve. I didn't set the step lengths.
$endgroup$
– feynman
Dec 13 '18 at 7:01
$begingroup$
How could we see what goes wrong in your calculus without without having your calculus in detail ?
$endgroup$
– JJacquelin
Dec 10 '18 at 14:25
$begingroup$
How could we see what goes wrong in your calculus without without having your calculus in detail ?
$endgroup$
– JJacquelin
Dec 10 '18 at 14:25
$begingroup$
Even for a simple quadratic function, gradient descent isn't guaranteed to converge if you don't pick a stepsize small enough. Consider for instance $f(x)=x^2$. If your stepsize is $h=1$, then $x_{n+1}=x_n-hf'(x_n)=x_n-2x_n=-x_n$ simply oscillates and never reaches the unique minimum.
$endgroup$
– Federico
Dec 10 '18 at 14:31
$begingroup$
Even for a simple quadratic function, gradient descent isn't guaranteed to converge if you don't pick a stepsize small enough. Consider for instance $f(x)=x^2$. If your stepsize is $h=1$, then $x_{n+1}=x_n-hf'(x_n)=x_n-2x_n=-x_n$ simply oscillates and never reaches the unique minimum.
$endgroup$
– Federico
Dec 10 '18 at 14:31
$begingroup$
@ JJacquelin I guarantee the rest of my calculus and gradient descent is correct. May you confirm that the above sum is correct?
$endgroup$
– feynman
Dec 13 '18 at 7:00
$begingroup$
@ JJacquelin I guarantee the rest of my calculus and gradient descent is correct. May you confirm that the above sum is correct?
$endgroup$
– feynman
Dec 13 '18 at 7:00
$begingroup$
@ Federico I guarantee my gradient descent calculation is correct, and step lengths are nothing to worry about because I use matlab ode23 package to solve. I didn't set the step lengths.
$endgroup$
– feynman
Dec 13 '18 at 7:01
$begingroup$
@ Federico I guarantee my gradient descent calculation is correct, and step lengths are nothing to worry about because I use matlab ode23 package to solve. I didn't set the step lengths.
$endgroup$
– feynman
Dec 13 '18 at 7:01
add a comment |
0
active
oldest
votes
Your Answer
StackExchange.ifUsing("editor", function () {
return StackExchange.using("mathjaxEditing", function () {
StackExchange.MarkdownEditor.creationCallbacks.add(function (editor, postfix) {
StackExchange.mathjaxEditing.prepareWmdForMathJax(editor, postfix, [["$", "$"], ["\\(","\\)"]]);
});
});
}, "mathjax-editing");
StackExchange.ready(function() {
var channelOptions = {
tags: "".split(" "),
id: "69"
};
initTagRenderer("".split(" "), "".split(" "), channelOptions);
StackExchange.using("externalEditor", function() {
// Have to fire editor after snippets, if snippets enabled
if (StackExchange.settings.snippets.snippetsEnabled) {
StackExchange.using("snippets", function() {
createEditor();
});
}
else {
createEditor();
}
});
function createEditor() {
StackExchange.prepareEditor({
heartbeatType: 'answer',
autoActivateHeartbeat: false,
convertImagesToLinks: true,
noModals: true,
showLowRepImageUploadWarning: true,
reputationToPostImages: 10,
bindNavPrevention: true,
postfix: "",
imageUploader: {
brandingHtml: "Powered by u003ca class="icon-imgur-white" href="https://imgur.com/"u003eu003c/au003e",
contentPolicyHtml: "User contributions licensed under u003ca href="https://creativecommons.org/licenses/by-sa/3.0/"u003ecc by-sa 3.0 with attribution requiredu003c/au003e u003ca href="https://stackoverflow.com/legal/content-policy"u003e(content policy)u003c/au003e",
allowUrls: true
},
noCode: true, onDemand: true,
discardSelector: ".discard-answer"
,immediatelyShowMarkdownHelp:true
});
}
});
Sign up or log in
StackExchange.ready(function () {
StackExchange.helpers.onClickDraftSave('#login-link');
});
Sign up using Google
Sign up using Facebook
Sign up using Email and Password
Post as a guest
Required, but never shown
StackExchange.ready(
function () {
StackExchange.openid.initPostLogin('.new-post-login', 'https%3a%2f%2fmath.stackexchange.com%2fquestions%2f3033958%2ftotal-least-square-fitting%23new-answer', 'question_page');
}
);
Post as a guest
Required, but never shown
0
active
oldest
votes
0
active
oldest
votes
active
oldest
votes
active
oldest
votes
Thanks for contributing an answer to Mathematics Stack Exchange!
- Please be sure to answer the question. Provide details and share your research!
But avoid …
- Asking for help, clarification, or responding to other answers.
- Making statements based on opinion; back them up with references or personal experience.
Use MathJax to format equations. MathJax reference.
To learn more, see our tips on writing great answers.
Sign up or log in
StackExchange.ready(function () {
StackExchange.helpers.onClickDraftSave('#login-link');
});
Sign up using Google
Sign up using Facebook
Sign up using Email and Password
Post as a guest
Required, but never shown
StackExchange.ready(
function () {
StackExchange.openid.initPostLogin('.new-post-login', 'https%3a%2f%2fmath.stackexchange.com%2fquestions%2f3033958%2ftotal-least-square-fitting%23new-answer', 'question_page');
}
);
Post as a guest
Required, but never shown
Sign up or log in
StackExchange.ready(function () {
StackExchange.helpers.onClickDraftSave('#login-link');
});
Sign up using Google
Sign up using Facebook
Sign up using Email and Password
Post as a guest
Required, but never shown
Sign up or log in
StackExchange.ready(function () {
StackExchange.helpers.onClickDraftSave('#login-link');
});
Sign up using Google
Sign up using Facebook
Sign up using Email and Password
Post as a guest
Required, but never shown
Sign up or log in
StackExchange.ready(function () {
StackExchange.helpers.onClickDraftSave('#login-link');
});
Sign up using Google
Sign up using Facebook
Sign up using Email and Password
Sign up using Google
Sign up using Facebook
Sign up using Email and Password
Post as a guest
Required, but never shown
Required, but never shown
Required, but never shown
Required, but never shown
Required, but never shown
Required, but never shown
Required, but never shown
Required, but never shown
Required, but never shown
0GWV8N82fqdohWtu0z1DtAy EmgTYI0dRMq7rorgHMJuqOFZ4,R wm9cV9wqd sM Sqrw jb ZyrGQM0rYtx1vnlPdJR
$begingroup$
How could we see what goes wrong in your calculus without without having your calculus in detail ?
$endgroup$
– JJacquelin
Dec 10 '18 at 14:25
$begingroup$
Even for a simple quadratic function, gradient descent isn't guaranteed to converge if you don't pick a stepsize small enough. Consider for instance $f(x)=x^2$. If your stepsize is $h=1$, then $x_{n+1}=x_n-hf'(x_n)=x_n-2x_n=-x_n$ simply oscillates and never reaches the unique minimum.
$endgroup$
– Federico
Dec 10 '18 at 14:31
$begingroup$
@ JJacquelin I guarantee the rest of my calculus and gradient descent is correct. May you confirm that the above sum is correct?
$endgroup$
– feynman
Dec 13 '18 at 7:00
$begingroup$
@ Federico I guarantee my gradient descent calculation is correct, and step lengths are nothing to worry about because I use matlab ode23 package to solve. I didn't set the step lengths.
$endgroup$
– feynman
Dec 13 '18 at 7:01