How to minimize the minimum mean square error of this difference
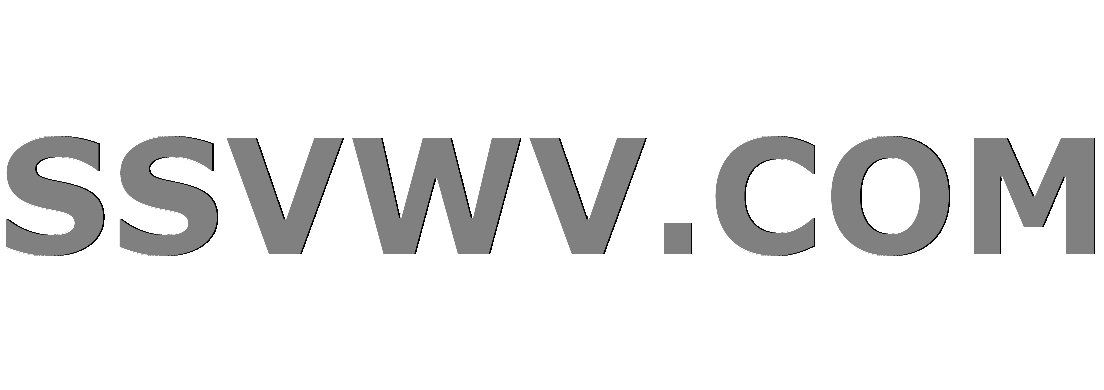
Multi tool use
$begingroup$
I am trying to minimize the mean square error. More precisely, I am trying to minimize the following optimization problem
$$arg min _{bf{w_1},bf{w_2}}mathbb{E} ,,[|{bf s} - {bf Wy}|^2 ]$$
$${bf W} = begin{bmatrix}
{bf w_1} &{bf 0 } \
{bf 0 } & {bf w_2 }
end{bmatrix}$$
where ${bf W}$ is a $2times N$ matrix and where ${bf w_i}$ is a $1times N/2$ for $iin[1:2]$ and ${bf 0}$ is $1times N/2$ vector while ${bf s}$ is 2 $times $ 1 and ${bf y}$ is $N times 1$ vectors.
Any hints or ideas on finding the minimizing vectors of this problem?
It is also given that
$${bf y = A F s + z}$$ where ${bf A}$ is $Ntimes N$ matrix while
$${bf F} = begin{bmatrix}
{bf f_1} &{bf 0} \
{bf 0} & {bf f_2}
end{bmatrix}$$
where ${bf f_i}$ are $N/2times 1$ vector and ${bf Z}$ is $Ntimes 1$ vector
I am thinking of starting to start the solution as following
$$argmin _{bf{w_1},bf{w_2}}mathbb{E} ,,[[{bf s} - {bf Wy}]^H[{bf s} - {bf Wy}]]$$
But I assume given the specific block diagonal structure of matrix ${bf W}$ and ${bf F}$ it should be easier to solve ...
Thanks
optimization mean-square-error
$endgroup$
add a comment |
$begingroup$
I am trying to minimize the mean square error. More precisely, I am trying to minimize the following optimization problem
$$arg min _{bf{w_1},bf{w_2}}mathbb{E} ,,[|{bf s} - {bf Wy}|^2 ]$$
$${bf W} = begin{bmatrix}
{bf w_1} &{bf 0 } \
{bf 0 } & {bf w_2 }
end{bmatrix}$$
where ${bf W}$ is a $2times N$ matrix and where ${bf w_i}$ is a $1times N/2$ for $iin[1:2]$ and ${bf 0}$ is $1times N/2$ vector while ${bf s}$ is 2 $times $ 1 and ${bf y}$ is $N times 1$ vectors.
Any hints or ideas on finding the minimizing vectors of this problem?
It is also given that
$${bf y = A F s + z}$$ where ${bf A}$ is $Ntimes N$ matrix while
$${bf F} = begin{bmatrix}
{bf f_1} &{bf 0} \
{bf 0} & {bf f_2}
end{bmatrix}$$
where ${bf f_i}$ are $N/2times 1$ vector and ${bf Z}$ is $Ntimes 1$ vector
I am thinking of starting to start the solution as following
$$argmin _{bf{w_1},bf{w_2}}mathbb{E} ,,[[{bf s} - {bf Wy}]^H[{bf s} - {bf Wy}]]$$
But I assume given the specific block diagonal structure of matrix ${bf W}$ and ${bf F}$ it should be easier to solve ...
Thanks
optimization mean-square-error
$endgroup$
$begingroup$
There must be something wrong with the dimensions. $mathbf W$ is a $2times N$-matrix and $mathbf y$ is a $2times 1$-vector, Then $mathbf{ Wy}$ is not possible.
$endgroup$
– callculus
Oct 22 '15 at 0:17
$begingroup$
sorry had typo thanks
$endgroup$
– Tyrone
Oct 22 '15 at 0:19
$begingroup$
I hope I´m not wrong, but it seems very similar to linear regression. Here it is $(s-Wy)'(s-Wy)=(s'-y'W')(s-Wy)=s's-s'Wy-y'W's-y'W'Wy$ But at linear regression it is optimized w.r.t $y$, not $W$. But I think it wouldn´t be bad to have a look on this subject.
$endgroup$
– callculus
Oct 22 '15 at 0:43
$begingroup$
thnk you for the reference
$endgroup$
– Tyrone
Oct 22 '15 at 1:41
add a comment |
$begingroup$
I am trying to minimize the mean square error. More precisely, I am trying to minimize the following optimization problem
$$arg min _{bf{w_1},bf{w_2}}mathbb{E} ,,[|{bf s} - {bf Wy}|^2 ]$$
$${bf W} = begin{bmatrix}
{bf w_1} &{bf 0 } \
{bf 0 } & {bf w_2 }
end{bmatrix}$$
where ${bf W}$ is a $2times N$ matrix and where ${bf w_i}$ is a $1times N/2$ for $iin[1:2]$ and ${bf 0}$ is $1times N/2$ vector while ${bf s}$ is 2 $times $ 1 and ${bf y}$ is $N times 1$ vectors.
Any hints or ideas on finding the minimizing vectors of this problem?
It is also given that
$${bf y = A F s + z}$$ where ${bf A}$ is $Ntimes N$ matrix while
$${bf F} = begin{bmatrix}
{bf f_1} &{bf 0} \
{bf 0} & {bf f_2}
end{bmatrix}$$
where ${bf f_i}$ are $N/2times 1$ vector and ${bf Z}$ is $Ntimes 1$ vector
I am thinking of starting to start the solution as following
$$argmin _{bf{w_1},bf{w_2}}mathbb{E} ,,[[{bf s} - {bf Wy}]^H[{bf s} - {bf Wy}]]$$
But I assume given the specific block diagonal structure of matrix ${bf W}$ and ${bf F}$ it should be easier to solve ...
Thanks
optimization mean-square-error
$endgroup$
I am trying to minimize the mean square error. More precisely, I am trying to minimize the following optimization problem
$$arg min _{bf{w_1},bf{w_2}}mathbb{E} ,,[|{bf s} - {bf Wy}|^2 ]$$
$${bf W} = begin{bmatrix}
{bf w_1} &{bf 0 } \
{bf 0 } & {bf w_2 }
end{bmatrix}$$
where ${bf W}$ is a $2times N$ matrix and where ${bf w_i}$ is a $1times N/2$ for $iin[1:2]$ and ${bf 0}$ is $1times N/2$ vector while ${bf s}$ is 2 $times $ 1 and ${bf y}$ is $N times 1$ vectors.
Any hints or ideas on finding the minimizing vectors of this problem?
It is also given that
$${bf y = A F s + z}$$ where ${bf A}$ is $Ntimes N$ matrix while
$${bf F} = begin{bmatrix}
{bf f_1} &{bf 0} \
{bf 0} & {bf f_2}
end{bmatrix}$$
where ${bf f_i}$ are $N/2times 1$ vector and ${bf Z}$ is $Ntimes 1$ vector
I am thinking of starting to start the solution as following
$$argmin _{bf{w_1},bf{w_2}}mathbb{E} ,,[[{bf s} - {bf Wy}]^H[{bf s} - {bf Wy}]]$$
But I assume given the specific block diagonal structure of matrix ${bf W}$ and ${bf F}$ it should be easier to solve ...
Thanks
optimization mean-square-error
optimization mean-square-error
edited Oct 22 '15 at 3:00
Tyrone
asked Oct 21 '15 at 23:46
TyroneTyrone
244418
244418
$begingroup$
There must be something wrong with the dimensions. $mathbf W$ is a $2times N$-matrix and $mathbf y$ is a $2times 1$-vector, Then $mathbf{ Wy}$ is not possible.
$endgroup$
– callculus
Oct 22 '15 at 0:17
$begingroup$
sorry had typo thanks
$endgroup$
– Tyrone
Oct 22 '15 at 0:19
$begingroup$
I hope I´m not wrong, but it seems very similar to linear regression. Here it is $(s-Wy)'(s-Wy)=(s'-y'W')(s-Wy)=s's-s'Wy-y'W's-y'W'Wy$ But at linear regression it is optimized w.r.t $y$, not $W$. But I think it wouldn´t be bad to have a look on this subject.
$endgroup$
– callculus
Oct 22 '15 at 0:43
$begingroup$
thnk you for the reference
$endgroup$
– Tyrone
Oct 22 '15 at 1:41
add a comment |
$begingroup$
There must be something wrong with the dimensions. $mathbf W$ is a $2times N$-matrix and $mathbf y$ is a $2times 1$-vector, Then $mathbf{ Wy}$ is not possible.
$endgroup$
– callculus
Oct 22 '15 at 0:17
$begingroup$
sorry had typo thanks
$endgroup$
– Tyrone
Oct 22 '15 at 0:19
$begingroup$
I hope I´m not wrong, but it seems very similar to linear regression. Here it is $(s-Wy)'(s-Wy)=(s'-y'W')(s-Wy)=s's-s'Wy-y'W's-y'W'Wy$ But at linear regression it is optimized w.r.t $y$, not $W$. But I think it wouldn´t be bad to have a look on this subject.
$endgroup$
– callculus
Oct 22 '15 at 0:43
$begingroup$
thnk you for the reference
$endgroup$
– Tyrone
Oct 22 '15 at 1:41
$begingroup$
There must be something wrong with the dimensions. $mathbf W$ is a $2times N$-matrix and $mathbf y$ is a $2times 1$-vector, Then $mathbf{ Wy}$ is not possible.
$endgroup$
– callculus
Oct 22 '15 at 0:17
$begingroup$
There must be something wrong with the dimensions. $mathbf W$ is a $2times N$-matrix and $mathbf y$ is a $2times 1$-vector, Then $mathbf{ Wy}$ is not possible.
$endgroup$
– callculus
Oct 22 '15 at 0:17
$begingroup$
sorry had typo thanks
$endgroup$
– Tyrone
Oct 22 '15 at 0:19
$begingroup$
sorry had typo thanks
$endgroup$
– Tyrone
Oct 22 '15 at 0:19
$begingroup$
I hope I´m not wrong, but it seems very similar to linear regression. Here it is $(s-Wy)'(s-Wy)=(s'-y'W')(s-Wy)=s's-s'Wy-y'W's-y'W'Wy$ But at linear regression it is optimized w.r.t $y$, not $W$. But I think it wouldn´t be bad to have a look on this subject.
$endgroup$
– callculus
Oct 22 '15 at 0:43
$begingroup$
I hope I´m not wrong, but it seems very similar to linear regression. Here it is $(s-Wy)'(s-Wy)=(s'-y'W')(s-Wy)=s's-s'Wy-y'W's-y'W'Wy$ But at linear regression it is optimized w.r.t $y$, not $W$. But I think it wouldn´t be bad to have a look on this subject.
$endgroup$
– callculus
Oct 22 '15 at 0:43
$begingroup$
thnk you for the reference
$endgroup$
– Tyrone
Oct 22 '15 at 1:41
$begingroup$
thnk you for the reference
$endgroup$
– Tyrone
Oct 22 '15 at 1:41
add a comment |
1 Answer
1
active
oldest
votes
$begingroup$
I assume you wish to find the non random matrix $W$ and hence its elements are not boldface in my solution. $mathbf s$ and $mathbf y$ are random vectors, hence they are boldface. First let me write the matrix ${W}
= begin{bmatrix}
{w_{1}^*} &{ 0 } \
{ 0 } & { w_{2}^* }
end{bmatrix}
$
where $*$ denotes the hermitian operation. Now all lower case vectors are column vectors.
This problem can be restated as
$arg min _{{w_1},{w_2}}mathbb{E} ,,left[leftVertbegin{bmatrix}
bf s_1^* \
bf s_{2}^*
end{bmatrix} - begin{bmatrix}
{bf y_{1}^*} &{bf 0 } \
{bf 0 } & {bf y_{2}^* }
end{bmatrix}begin{bmatrix}
w_1 \
w_{2}
end{bmatrix}rightVert^2right] $.
After this, the problem decouples to solving for $w_1$ and $w_2$. For eg. for $w_1$, we need to minimize $J =mathbb E[ ||mathbf s_1^* - mathbf y_1^* w_1||^2]$.
Just expand the inside argument and differentiate w.r.t. $w_1^*$ and put the gradient to $0$. We get the solution for $w_1$ as
$mathbb E [mathbf y_1 mathbf y_1^*] w_1 = mathbb E [mathbf y_1^* mathbf s_1^*]$.
Now, assuming you can find the correlation matrix of $mathbf y_1$ and it is invertible, and the cross correlation between $mathbf y_1$ and $mathbf s_1$, you can find $w_1$. Similarly, you can solve for $w_2$.
$endgroup$
$begingroup$
i am not sure... wht how did you decompose vector y?
$endgroup$
– Tyrone
Oct 22 '15 at 1:49
$begingroup$
The Nx1 vector $mathbf y$ is split into two vectors $mathbf y_1$ and $mathbf y_2$ each N/2 x1, stacked on top of each other. Hope that clears the confusion.
$endgroup$
– shaktiman
Oct 22 '15 at 3:31
add a comment |
Your Answer
StackExchange.ifUsing("editor", function () {
return StackExchange.using("mathjaxEditing", function () {
StackExchange.MarkdownEditor.creationCallbacks.add(function (editor, postfix) {
StackExchange.mathjaxEditing.prepareWmdForMathJax(editor, postfix, [["$", "$"], ["\\(","\\)"]]);
});
});
}, "mathjax-editing");
StackExchange.ready(function() {
var channelOptions = {
tags: "".split(" "),
id: "69"
};
initTagRenderer("".split(" "), "".split(" "), channelOptions);
StackExchange.using("externalEditor", function() {
// Have to fire editor after snippets, if snippets enabled
if (StackExchange.settings.snippets.snippetsEnabled) {
StackExchange.using("snippets", function() {
createEditor();
});
}
else {
createEditor();
}
});
function createEditor() {
StackExchange.prepareEditor({
heartbeatType: 'answer',
autoActivateHeartbeat: false,
convertImagesToLinks: true,
noModals: true,
showLowRepImageUploadWarning: true,
reputationToPostImages: 10,
bindNavPrevention: true,
postfix: "",
imageUploader: {
brandingHtml: "Powered by u003ca class="icon-imgur-white" href="https://imgur.com/"u003eu003c/au003e",
contentPolicyHtml: "User contributions licensed under u003ca href="https://creativecommons.org/licenses/by-sa/3.0/"u003ecc by-sa 3.0 with attribution requiredu003c/au003e u003ca href="https://stackoverflow.com/legal/content-policy"u003e(content policy)u003c/au003e",
allowUrls: true
},
noCode: true, onDemand: true,
discardSelector: ".discard-answer"
,immediatelyShowMarkdownHelp:true
});
}
});
Sign up or log in
StackExchange.ready(function () {
StackExchange.helpers.onClickDraftSave('#login-link');
});
Sign up using Google
Sign up using Facebook
Sign up using Email and Password
Post as a guest
Required, but never shown
StackExchange.ready(
function () {
StackExchange.openid.initPostLogin('.new-post-login', 'https%3a%2f%2fmath.stackexchange.com%2fquestions%2f1491594%2fhow-to-minimize-the-minimum-mean-square-error-of-this-difference%23new-answer', 'question_page');
}
);
Post as a guest
Required, but never shown
1 Answer
1
active
oldest
votes
1 Answer
1
active
oldest
votes
active
oldest
votes
active
oldest
votes
$begingroup$
I assume you wish to find the non random matrix $W$ and hence its elements are not boldface in my solution. $mathbf s$ and $mathbf y$ are random vectors, hence they are boldface. First let me write the matrix ${W}
= begin{bmatrix}
{w_{1}^*} &{ 0 } \
{ 0 } & { w_{2}^* }
end{bmatrix}
$
where $*$ denotes the hermitian operation. Now all lower case vectors are column vectors.
This problem can be restated as
$arg min _{{w_1},{w_2}}mathbb{E} ,,left[leftVertbegin{bmatrix}
bf s_1^* \
bf s_{2}^*
end{bmatrix} - begin{bmatrix}
{bf y_{1}^*} &{bf 0 } \
{bf 0 } & {bf y_{2}^* }
end{bmatrix}begin{bmatrix}
w_1 \
w_{2}
end{bmatrix}rightVert^2right] $.
After this, the problem decouples to solving for $w_1$ and $w_2$. For eg. for $w_1$, we need to minimize $J =mathbb E[ ||mathbf s_1^* - mathbf y_1^* w_1||^2]$.
Just expand the inside argument and differentiate w.r.t. $w_1^*$ and put the gradient to $0$. We get the solution for $w_1$ as
$mathbb E [mathbf y_1 mathbf y_1^*] w_1 = mathbb E [mathbf y_1^* mathbf s_1^*]$.
Now, assuming you can find the correlation matrix of $mathbf y_1$ and it is invertible, and the cross correlation between $mathbf y_1$ and $mathbf s_1$, you can find $w_1$. Similarly, you can solve for $w_2$.
$endgroup$
$begingroup$
i am not sure... wht how did you decompose vector y?
$endgroup$
– Tyrone
Oct 22 '15 at 1:49
$begingroup$
The Nx1 vector $mathbf y$ is split into two vectors $mathbf y_1$ and $mathbf y_2$ each N/2 x1, stacked on top of each other. Hope that clears the confusion.
$endgroup$
– shaktiman
Oct 22 '15 at 3:31
add a comment |
$begingroup$
I assume you wish to find the non random matrix $W$ and hence its elements are not boldface in my solution. $mathbf s$ and $mathbf y$ are random vectors, hence they are boldface. First let me write the matrix ${W}
= begin{bmatrix}
{w_{1}^*} &{ 0 } \
{ 0 } & { w_{2}^* }
end{bmatrix}
$
where $*$ denotes the hermitian operation. Now all lower case vectors are column vectors.
This problem can be restated as
$arg min _{{w_1},{w_2}}mathbb{E} ,,left[leftVertbegin{bmatrix}
bf s_1^* \
bf s_{2}^*
end{bmatrix} - begin{bmatrix}
{bf y_{1}^*} &{bf 0 } \
{bf 0 } & {bf y_{2}^* }
end{bmatrix}begin{bmatrix}
w_1 \
w_{2}
end{bmatrix}rightVert^2right] $.
After this, the problem decouples to solving for $w_1$ and $w_2$. For eg. for $w_1$, we need to minimize $J =mathbb E[ ||mathbf s_1^* - mathbf y_1^* w_1||^2]$.
Just expand the inside argument and differentiate w.r.t. $w_1^*$ and put the gradient to $0$. We get the solution for $w_1$ as
$mathbb E [mathbf y_1 mathbf y_1^*] w_1 = mathbb E [mathbf y_1^* mathbf s_1^*]$.
Now, assuming you can find the correlation matrix of $mathbf y_1$ and it is invertible, and the cross correlation between $mathbf y_1$ and $mathbf s_1$, you can find $w_1$. Similarly, you can solve for $w_2$.
$endgroup$
$begingroup$
i am not sure... wht how did you decompose vector y?
$endgroup$
– Tyrone
Oct 22 '15 at 1:49
$begingroup$
The Nx1 vector $mathbf y$ is split into two vectors $mathbf y_1$ and $mathbf y_2$ each N/2 x1, stacked on top of each other. Hope that clears the confusion.
$endgroup$
– shaktiman
Oct 22 '15 at 3:31
add a comment |
$begingroup$
I assume you wish to find the non random matrix $W$ and hence its elements are not boldface in my solution. $mathbf s$ and $mathbf y$ are random vectors, hence they are boldface. First let me write the matrix ${W}
= begin{bmatrix}
{w_{1}^*} &{ 0 } \
{ 0 } & { w_{2}^* }
end{bmatrix}
$
where $*$ denotes the hermitian operation. Now all lower case vectors are column vectors.
This problem can be restated as
$arg min _{{w_1},{w_2}}mathbb{E} ,,left[leftVertbegin{bmatrix}
bf s_1^* \
bf s_{2}^*
end{bmatrix} - begin{bmatrix}
{bf y_{1}^*} &{bf 0 } \
{bf 0 } & {bf y_{2}^* }
end{bmatrix}begin{bmatrix}
w_1 \
w_{2}
end{bmatrix}rightVert^2right] $.
After this, the problem decouples to solving for $w_1$ and $w_2$. For eg. for $w_1$, we need to minimize $J =mathbb E[ ||mathbf s_1^* - mathbf y_1^* w_1||^2]$.
Just expand the inside argument and differentiate w.r.t. $w_1^*$ and put the gradient to $0$. We get the solution for $w_1$ as
$mathbb E [mathbf y_1 mathbf y_1^*] w_1 = mathbb E [mathbf y_1^* mathbf s_1^*]$.
Now, assuming you can find the correlation matrix of $mathbf y_1$ and it is invertible, and the cross correlation between $mathbf y_1$ and $mathbf s_1$, you can find $w_1$. Similarly, you can solve for $w_2$.
$endgroup$
I assume you wish to find the non random matrix $W$ and hence its elements are not boldface in my solution. $mathbf s$ and $mathbf y$ are random vectors, hence they are boldface. First let me write the matrix ${W}
= begin{bmatrix}
{w_{1}^*} &{ 0 } \
{ 0 } & { w_{2}^* }
end{bmatrix}
$
where $*$ denotes the hermitian operation. Now all lower case vectors are column vectors.
This problem can be restated as
$arg min _{{w_1},{w_2}}mathbb{E} ,,left[leftVertbegin{bmatrix}
bf s_1^* \
bf s_{2}^*
end{bmatrix} - begin{bmatrix}
{bf y_{1}^*} &{bf 0 } \
{bf 0 } & {bf y_{2}^* }
end{bmatrix}begin{bmatrix}
w_1 \
w_{2}
end{bmatrix}rightVert^2right] $.
After this, the problem decouples to solving for $w_1$ and $w_2$. For eg. for $w_1$, we need to minimize $J =mathbb E[ ||mathbf s_1^* - mathbf y_1^* w_1||^2]$.
Just expand the inside argument and differentiate w.r.t. $w_1^*$ and put the gradient to $0$. We get the solution for $w_1$ as
$mathbb E [mathbf y_1 mathbf y_1^*] w_1 = mathbb E [mathbf y_1^* mathbf s_1^*]$.
Now, assuming you can find the correlation matrix of $mathbf y_1$ and it is invertible, and the cross correlation between $mathbf y_1$ and $mathbf s_1$, you can find $w_1$. Similarly, you can solve for $w_2$.
answered Oct 22 '15 at 0:29
shaktimanshaktiman
628
628
$begingroup$
i am not sure... wht how did you decompose vector y?
$endgroup$
– Tyrone
Oct 22 '15 at 1:49
$begingroup$
The Nx1 vector $mathbf y$ is split into two vectors $mathbf y_1$ and $mathbf y_2$ each N/2 x1, stacked on top of each other. Hope that clears the confusion.
$endgroup$
– shaktiman
Oct 22 '15 at 3:31
add a comment |
$begingroup$
i am not sure... wht how did you decompose vector y?
$endgroup$
– Tyrone
Oct 22 '15 at 1:49
$begingroup$
The Nx1 vector $mathbf y$ is split into two vectors $mathbf y_1$ and $mathbf y_2$ each N/2 x1, stacked on top of each other. Hope that clears the confusion.
$endgroup$
– shaktiman
Oct 22 '15 at 3:31
$begingroup$
i am not sure... wht how did you decompose vector y?
$endgroup$
– Tyrone
Oct 22 '15 at 1:49
$begingroup$
i am not sure... wht how did you decompose vector y?
$endgroup$
– Tyrone
Oct 22 '15 at 1:49
$begingroup$
The Nx1 vector $mathbf y$ is split into two vectors $mathbf y_1$ and $mathbf y_2$ each N/2 x1, stacked on top of each other. Hope that clears the confusion.
$endgroup$
– shaktiman
Oct 22 '15 at 3:31
$begingroup$
The Nx1 vector $mathbf y$ is split into two vectors $mathbf y_1$ and $mathbf y_2$ each N/2 x1, stacked on top of each other. Hope that clears the confusion.
$endgroup$
– shaktiman
Oct 22 '15 at 3:31
add a comment |
Thanks for contributing an answer to Mathematics Stack Exchange!
- Please be sure to answer the question. Provide details and share your research!
But avoid …
- Asking for help, clarification, or responding to other answers.
- Making statements based on opinion; back them up with references or personal experience.
Use MathJax to format equations. MathJax reference.
To learn more, see our tips on writing great answers.
Sign up or log in
StackExchange.ready(function () {
StackExchange.helpers.onClickDraftSave('#login-link');
});
Sign up using Google
Sign up using Facebook
Sign up using Email and Password
Post as a guest
Required, but never shown
StackExchange.ready(
function () {
StackExchange.openid.initPostLogin('.new-post-login', 'https%3a%2f%2fmath.stackexchange.com%2fquestions%2f1491594%2fhow-to-minimize-the-minimum-mean-square-error-of-this-difference%23new-answer', 'question_page');
}
);
Post as a guest
Required, but never shown
Sign up or log in
StackExchange.ready(function () {
StackExchange.helpers.onClickDraftSave('#login-link');
});
Sign up using Google
Sign up using Facebook
Sign up using Email and Password
Post as a guest
Required, but never shown
Sign up or log in
StackExchange.ready(function () {
StackExchange.helpers.onClickDraftSave('#login-link');
});
Sign up using Google
Sign up using Facebook
Sign up using Email and Password
Post as a guest
Required, but never shown
Sign up or log in
StackExchange.ready(function () {
StackExchange.helpers.onClickDraftSave('#login-link');
});
Sign up using Google
Sign up using Facebook
Sign up using Email and Password
Sign up using Google
Sign up using Facebook
Sign up using Email and Password
Post as a guest
Required, but never shown
Required, but never shown
Required, but never shown
Required, but never shown
Required, but never shown
Required, but never shown
Required, but never shown
Required, but never shown
Required, but never shown
Ldi MBnMbz0plXvDC7d w,7LW,K YdqWplhsF6kX HH4u66aH,gs,iZ,C24DpBh6JqqsbdB7Ar6MjZy,XrbjX t,tve Kz2
$begingroup$
There must be something wrong with the dimensions. $mathbf W$ is a $2times N$-matrix and $mathbf y$ is a $2times 1$-vector, Then $mathbf{ Wy}$ is not possible.
$endgroup$
– callculus
Oct 22 '15 at 0:17
$begingroup$
sorry had typo thanks
$endgroup$
– Tyrone
Oct 22 '15 at 0:19
$begingroup$
I hope I´m not wrong, but it seems very similar to linear regression. Here it is $(s-Wy)'(s-Wy)=(s'-y'W')(s-Wy)=s's-s'Wy-y'W's-y'W'Wy$ But at linear regression it is optimized w.r.t $y$, not $W$. But I think it wouldn´t be bad to have a look on this subject.
$endgroup$
– callculus
Oct 22 '15 at 0:43
$begingroup$
thnk you for the reference
$endgroup$
– Tyrone
Oct 22 '15 at 1:41