How to handle optimization problems when optimization variable is matrix?
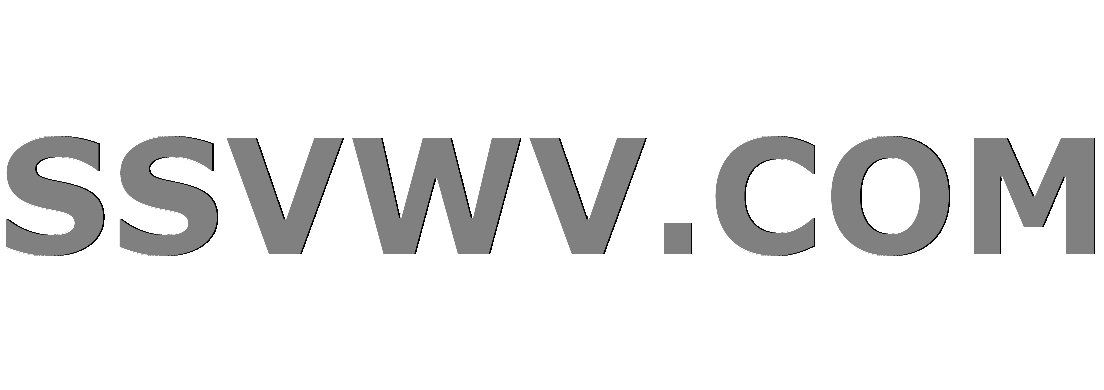
Multi tool use
$begingroup$
Suppose we have the following optimization problem
$$
min_{0preceq M preceq I} y^TMy
$$
where $y in mathbb{R}^n$ and $M in mathbb{R}^{n times n}$ is a positive semi-definite matrix. Notice that the optimization variable is matrix.
Is there any algebraically way to handle this in terms of $M$?
When we write it as the standard form we have the following
$$
min y^TMy
$$
$$
text{s.t.},,,,,, {g_1(M)=-M preceq 0 }
$$
$$
text{s.t.},,,,,, {g_2(M)=I-M preceq 0 }
$$
which is a matrix inequality. If it were vector, it was doable but what would we do when they are in matrix form.
I want to write the first order optimality condition using Lagrangian in terms of gradient of $g_1(M)$ and $g_2(M)$ but the gradient in terms of $M$ is $I$ and $-I$.
The following answer explains it using different view.
What is the KKT condition for constraint $M preceq I$?
I want a method that ties these to views together.
I want to know the general case. I want to handle it directly in terms of M.
I will appreciate, If you introduce me any reference that addresses this issue.
optimization convex-optimization karush-kuhn-tucker
$endgroup$
add a comment |
$begingroup$
Suppose we have the following optimization problem
$$
min_{0preceq M preceq I} y^TMy
$$
where $y in mathbb{R}^n$ and $M in mathbb{R}^{n times n}$ is a positive semi-definite matrix. Notice that the optimization variable is matrix.
Is there any algebraically way to handle this in terms of $M$?
When we write it as the standard form we have the following
$$
min y^TMy
$$
$$
text{s.t.},,,,,, {g_1(M)=-M preceq 0 }
$$
$$
text{s.t.},,,,,, {g_2(M)=I-M preceq 0 }
$$
which is a matrix inequality. If it were vector, it was doable but what would we do when they are in matrix form.
I want to write the first order optimality condition using Lagrangian in terms of gradient of $g_1(M)$ and $g_2(M)$ but the gradient in terms of $M$ is $I$ and $-I$.
The following answer explains it using different view.
What is the KKT condition for constraint $M preceq I$?
I want a method that ties these to views together.
I want to know the general case. I want to handle it directly in terms of M.
I will appreciate, If you introduce me any reference that addresses this issue.
optimization convex-optimization karush-kuhn-tucker
$endgroup$
2
$begingroup$
Doesn't $M=0$ solve the problem?
$endgroup$
– A.Γ.
Dec 21 '18 at 17:29
add a comment |
$begingroup$
Suppose we have the following optimization problem
$$
min_{0preceq M preceq I} y^TMy
$$
where $y in mathbb{R}^n$ and $M in mathbb{R}^{n times n}$ is a positive semi-definite matrix. Notice that the optimization variable is matrix.
Is there any algebraically way to handle this in terms of $M$?
When we write it as the standard form we have the following
$$
min y^TMy
$$
$$
text{s.t.},,,,,, {g_1(M)=-M preceq 0 }
$$
$$
text{s.t.},,,,,, {g_2(M)=I-M preceq 0 }
$$
which is a matrix inequality. If it were vector, it was doable but what would we do when they are in matrix form.
I want to write the first order optimality condition using Lagrangian in terms of gradient of $g_1(M)$ and $g_2(M)$ but the gradient in terms of $M$ is $I$ and $-I$.
The following answer explains it using different view.
What is the KKT condition for constraint $M preceq I$?
I want a method that ties these to views together.
I want to know the general case. I want to handle it directly in terms of M.
I will appreciate, If you introduce me any reference that addresses this issue.
optimization convex-optimization karush-kuhn-tucker
$endgroup$
Suppose we have the following optimization problem
$$
min_{0preceq M preceq I} y^TMy
$$
where $y in mathbb{R}^n$ and $M in mathbb{R}^{n times n}$ is a positive semi-definite matrix. Notice that the optimization variable is matrix.
Is there any algebraically way to handle this in terms of $M$?
When we write it as the standard form we have the following
$$
min y^TMy
$$
$$
text{s.t.},,,,,, {g_1(M)=-M preceq 0 }
$$
$$
text{s.t.},,,,,, {g_2(M)=I-M preceq 0 }
$$
which is a matrix inequality. If it were vector, it was doable but what would we do when they are in matrix form.
I want to write the first order optimality condition using Lagrangian in terms of gradient of $g_1(M)$ and $g_2(M)$ but the gradient in terms of $M$ is $I$ and $-I$.
The following answer explains it using different view.
What is the KKT condition for constraint $M preceq I$?
I want a method that ties these to views together.
I want to know the general case. I want to handle it directly in terms of M.
I will appreciate, If you introduce me any reference that addresses this issue.
optimization convex-optimization karush-kuhn-tucker
optimization convex-optimization karush-kuhn-tucker
asked Dec 21 '18 at 17:27
SaeedSaeed
1,149310
1,149310
2
$begingroup$
Doesn't $M=0$ solve the problem?
$endgroup$
– A.Γ.
Dec 21 '18 at 17:29
add a comment |
2
$begingroup$
Doesn't $M=0$ solve the problem?
$endgroup$
– A.Γ.
Dec 21 '18 at 17:29
2
2
$begingroup$
Doesn't $M=0$ solve the problem?
$endgroup$
– A.Γ.
Dec 21 '18 at 17:29
$begingroup$
Doesn't $M=0$ solve the problem?
$endgroup$
– A.Γ.
Dec 21 '18 at 17:29
add a comment |
1 Answer
1
active
oldest
votes
$begingroup$
This topic is discussed in Convex Optimization by Boyd and Vandenberghe. See section 5.9.
The key idea here is that you need an appropriate inner product associated with the conic inequality. For positive semidefiniteness constraints, the associated inner product is $langle A, B rangle=mbox{tr}(A^{T}B)$. The Lagrange multiplier for the conic constraint must be a positive semidefinite matrix $Lambda succeq 0$ rather than a scalar. All of the theory for scalar constraints carries over in a straight-forward way to this more general setting.
$endgroup$
add a comment |
Your Answer
StackExchange.ready(function() {
var channelOptions = {
tags: "".split(" "),
id: "69"
};
initTagRenderer("".split(" "), "".split(" "), channelOptions);
StackExchange.using("externalEditor", function() {
// Have to fire editor after snippets, if snippets enabled
if (StackExchange.settings.snippets.snippetsEnabled) {
StackExchange.using("snippets", function() {
createEditor();
});
}
else {
createEditor();
}
});
function createEditor() {
StackExchange.prepareEditor({
heartbeatType: 'answer',
autoActivateHeartbeat: false,
convertImagesToLinks: true,
noModals: true,
showLowRepImageUploadWarning: true,
reputationToPostImages: 10,
bindNavPrevention: true,
postfix: "",
imageUploader: {
brandingHtml: "Powered by u003ca class="icon-imgur-white" href="https://imgur.com/"u003eu003c/au003e",
contentPolicyHtml: "User contributions licensed under u003ca href="https://creativecommons.org/licenses/by-sa/3.0/"u003ecc by-sa 3.0 with attribution requiredu003c/au003e u003ca href="https://stackoverflow.com/legal/content-policy"u003e(content policy)u003c/au003e",
allowUrls: true
},
noCode: true, onDemand: true,
discardSelector: ".discard-answer"
,immediatelyShowMarkdownHelp:true
});
}
});
Sign up or log in
StackExchange.ready(function () {
StackExchange.helpers.onClickDraftSave('#login-link');
});
Sign up using Google
Sign up using Facebook
Sign up using Email and Password
Post as a guest
Required, but never shown
StackExchange.ready(
function () {
StackExchange.openid.initPostLogin('.new-post-login', 'https%3a%2f%2fmath.stackexchange.com%2fquestions%2f3048722%2fhow-to-handle-optimization-problems-when-optimization-variable-is-matrix%23new-answer', 'question_page');
}
);
Post as a guest
Required, but never shown
1 Answer
1
active
oldest
votes
1 Answer
1
active
oldest
votes
active
oldest
votes
active
oldest
votes
$begingroup$
This topic is discussed in Convex Optimization by Boyd and Vandenberghe. See section 5.9.
The key idea here is that you need an appropriate inner product associated with the conic inequality. For positive semidefiniteness constraints, the associated inner product is $langle A, B rangle=mbox{tr}(A^{T}B)$. The Lagrange multiplier for the conic constraint must be a positive semidefinite matrix $Lambda succeq 0$ rather than a scalar. All of the theory for scalar constraints carries over in a straight-forward way to this more general setting.
$endgroup$
add a comment |
$begingroup$
This topic is discussed in Convex Optimization by Boyd and Vandenberghe. See section 5.9.
The key idea here is that you need an appropriate inner product associated with the conic inequality. For positive semidefiniteness constraints, the associated inner product is $langle A, B rangle=mbox{tr}(A^{T}B)$. The Lagrange multiplier for the conic constraint must be a positive semidefinite matrix $Lambda succeq 0$ rather than a scalar. All of the theory for scalar constraints carries over in a straight-forward way to this more general setting.
$endgroup$
add a comment |
$begingroup$
This topic is discussed in Convex Optimization by Boyd and Vandenberghe. See section 5.9.
The key idea here is that you need an appropriate inner product associated with the conic inequality. For positive semidefiniteness constraints, the associated inner product is $langle A, B rangle=mbox{tr}(A^{T}B)$. The Lagrange multiplier for the conic constraint must be a positive semidefinite matrix $Lambda succeq 0$ rather than a scalar. All of the theory for scalar constraints carries over in a straight-forward way to this more general setting.
$endgroup$
This topic is discussed in Convex Optimization by Boyd and Vandenberghe. See section 5.9.
The key idea here is that you need an appropriate inner product associated with the conic inequality. For positive semidefiniteness constraints, the associated inner product is $langle A, B rangle=mbox{tr}(A^{T}B)$. The Lagrange multiplier for the conic constraint must be a positive semidefinite matrix $Lambda succeq 0$ rather than a scalar. All of the theory for scalar constraints carries over in a straight-forward way to this more general setting.
answered Dec 21 '18 at 18:12
Brian BorchersBrian Borchers
6,29611320
6,29611320
add a comment |
add a comment |
Thanks for contributing an answer to Mathematics Stack Exchange!
- Please be sure to answer the question. Provide details and share your research!
But avoid …
- Asking for help, clarification, or responding to other answers.
- Making statements based on opinion; back them up with references or personal experience.
Use MathJax to format equations. MathJax reference.
To learn more, see our tips on writing great answers.
Sign up or log in
StackExchange.ready(function () {
StackExchange.helpers.onClickDraftSave('#login-link');
});
Sign up using Google
Sign up using Facebook
Sign up using Email and Password
Post as a guest
Required, but never shown
StackExchange.ready(
function () {
StackExchange.openid.initPostLogin('.new-post-login', 'https%3a%2f%2fmath.stackexchange.com%2fquestions%2f3048722%2fhow-to-handle-optimization-problems-when-optimization-variable-is-matrix%23new-answer', 'question_page');
}
);
Post as a guest
Required, but never shown
Sign up or log in
StackExchange.ready(function () {
StackExchange.helpers.onClickDraftSave('#login-link');
});
Sign up using Google
Sign up using Facebook
Sign up using Email and Password
Post as a guest
Required, but never shown
Sign up or log in
StackExchange.ready(function () {
StackExchange.helpers.onClickDraftSave('#login-link');
});
Sign up using Google
Sign up using Facebook
Sign up using Email and Password
Post as a guest
Required, but never shown
Sign up or log in
StackExchange.ready(function () {
StackExchange.helpers.onClickDraftSave('#login-link');
});
Sign up using Google
Sign up using Facebook
Sign up using Email and Password
Sign up using Google
Sign up using Facebook
Sign up using Email and Password
Post as a guest
Required, but never shown
Required, but never shown
Required, but never shown
Required, but never shown
Required, but never shown
Required, but never shown
Required, but never shown
Required, but never shown
Required, but never shown
QuSLc,LJ kfVouED3C7UmA gJsYTrNxb7dC97D KPXp0hCsDt kAXD5539lMZOYGcvmmOnBmku
2
$begingroup$
Doesn't $M=0$ solve the problem?
$endgroup$
– A.Γ.
Dec 21 '18 at 17:29