Nullity of a matrix transformation
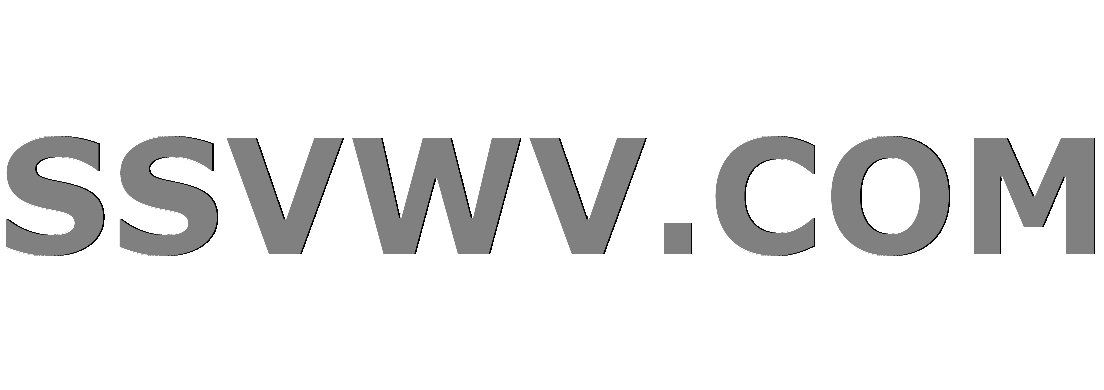
Multi tool use
$begingroup$
I'm having some trouble with one part of this question.
Which of the following statements are true?
$(1)$ For $thetaepsilonmathbb{R}$ fixed, $R:mathbb{R^2} rightarrow mathbb{R^2}, R(x,y)=(xcos(theta)-ysin(theta),xsin(theta)+ycos(theta))$ is linear.
$(2)$ The transformation $T:mathbb{R^2} rightarrow mathbb{R^2},T(x,y)=(1+x^2,1+y^2)$ is linear.
$(3)$ For $ngeq1$, every linear transformation $L:R:mathbb{R}^n rightarrow mathbb{R}^n$ satisties $L(mathbf{0})=mathbf{0}$.
$(4)$ If $L:mathbb{R^3} rightarrow mathbb{R^2}$ is linear, then the matrix which represents $L$ with respect to the standard coordinates must have positive nullity.
$(5)$ If $L:mathbb{R^2} rightarrow mathbb{R^3}$ is linear, then the matrix which represents $L$ with respect to the standard coordinates must have positive nullity.
$(A)$ Only $(1)$,$(3)$, and $(5)$ are true.
$(B)$ Only $(1)$,$(3)$, and $(4)$ are true.
$(C)$ Only $(2)$,$(3)$, and $(4)$ are true.
$(D)$ Only $(2)$,$(3)$, and $(5)$ are true.
$(E)$ Only $(1)$,$(2)$, and $(5)$ are true.
I know that $(1)$ is true, $(2)$ is false and $(3)$ is true. What is the difference between $(4)$ and $(5)$ though? How do I know which one is correct? Apparently statement $(4)$ is the correct one but why is that?
linear-algebra linear-transformations
$endgroup$
add a comment |
$begingroup$
I'm having some trouble with one part of this question.
Which of the following statements are true?
$(1)$ For $thetaepsilonmathbb{R}$ fixed, $R:mathbb{R^2} rightarrow mathbb{R^2}, R(x,y)=(xcos(theta)-ysin(theta),xsin(theta)+ycos(theta))$ is linear.
$(2)$ The transformation $T:mathbb{R^2} rightarrow mathbb{R^2},T(x,y)=(1+x^2,1+y^2)$ is linear.
$(3)$ For $ngeq1$, every linear transformation $L:R:mathbb{R}^n rightarrow mathbb{R}^n$ satisties $L(mathbf{0})=mathbf{0}$.
$(4)$ If $L:mathbb{R^3} rightarrow mathbb{R^2}$ is linear, then the matrix which represents $L$ with respect to the standard coordinates must have positive nullity.
$(5)$ If $L:mathbb{R^2} rightarrow mathbb{R^3}$ is linear, then the matrix which represents $L$ with respect to the standard coordinates must have positive nullity.
$(A)$ Only $(1)$,$(3)$, and $(5)$ are true.
$(B)$ Only $(1)$,$(3)$, and $(4)$ are true.
$(C)$ Only $(2)$,$(3)$, and $(4)$ are true.
$(D)$ Only $(2)$,$(3)$, and $(5)$ are true.
$(E)$ Only $(1)$,$(2)$, and $(5)$ are true.
I know that $(1)$ is true, $(2)$ is false and $(3)$ is true. What is the difference between $(4)$ and $(5)$ though? How do I know which one is correct? Apparently statement $(4)$ is the correct one but why is that?
linear-algebra linear-transformations
$endgroup$
$begingroup$
Hint: think about the rank-nullity theorem.
$endgroup$
– angryavian
Dec 23 '18 at 5:00
$begingroup$
How? I need to know what the rank is and the number of columns to be useful.
$endgroup$
– Future Math person
Dec 23 '18 at 5:02
$begingroup$
Doesn't $mathbb{R}^3 to mathbb{R}^2$ tell you about the shape of the matrix?
$endgroup$
– angryavian
Dec 23 '18 at 5:03
$begingroup$
It does but I'm confused if the number of columns would be 2 or 3. Would it be 2 because that's what I have transformed to?
$endgroup$
– Future Math person
Dec 23 '18 at 5:04
$begingroup$
If $M$ is the matrix and $v in mathbb{R}^3$ then the result of applying $L$ to $v$ is $Mv$.
$endgroup$
– angryavian
Dec 23 '18 at 5:06
add a comment |
$begingroup$
I'm having some trouble with one part of this question.
Which of the following statements are true?
$(1)$ For $thetaepsilonmathbb{R}$ fixed, $R:mathbb{R^2} rightarrow mathbb{R^2}, R(x,y)=(xcos(theta)-ysin(theta),xsin(theta)+ycos(theta))$ is linear.
$(2)$ The transformation $T:mathbb{R^2} rightarrow mathbb{R^2},T(x,y)=(1+x^2,1+y^2)$ is linear.
$(3)$ For $ngeq1$, every linear transformation $L:R:mathbb{R}^n rightarrow mathbb{R}^n$ satisties $L(mathbf{0})=mathbf{0}$.
$(4)$ If $L:mathbb{R^3} rightarrow mathbb{R^2}$ is linear, then the matrix which represents $L$ with respect to the standard coordinates must have positive nullity.
$(5)$ If $L:mathbb{R^2} rightarrow mathbb{R^3}$ is linear, then the matrix which represents $L$ with respect to the standard coordinates must have positive nullity.
$(A)$ Only $(1)$,$(3)$, and $(5)$ are true.
$(B)$ Only $(1)$,$(3)$, and $(4)$ are true.
$(C)$ Only $(2)$,$(3)$, and $(4)$ are true.
$(D)$ Only $(2)$,$(3)$, and $(5)$ are true.
$(E)$ Only $(1)$,$(2)$, and $(5)$ are true.
I know that $(1)$ is true, $(2)$ is false and $(3)$ is true. What is the difference between $(4)$ and $(5)$ though? How do I know which one is correct? Apparently statement $(4)$ is the correct one but why is that?
linear-algebra linear-transformations
$endgroup$
I'm having some trouble with one part of this question.
Which of the following statements are true?
$(1)$ For $thetaepsilonmathbb{R}$ fixed, $R:mathbb{R^2} rightarrow mathbb{R^2}, R(x,y)=(xcos(theta)-ysin(theta),xsin(theta)+ycos(theta))$ is linear.
$(2)$ The transformation $T:mathbb{R^2} rightarrow mathbb{R^2},T(x,y)=(1+x^2,1+y^2)$ is linear.
$(3)$ For $ngeq1$, every linear transformation $L:R:mathbb{R}^n rightarrow mathbb{R}^n$ satisties $L(mathbf{0})=mathbf{0}$.
$(4)$ If $L:mathbb{R^3} rightarrow mathbb{R^2}$ is linear, then the matrix which represents $L$ with respect to the standard coordinates must have positive nullity.
$(5)$ If $L:mathbb{R^2} rightarrow mathbb{R^3}$ is linear, then the matrix which represents $L$ with respect to the standard coordinates must have positive nullity.
$(A)$ Only $(1)$,$(3)$, and $(5)$ are true.
$(B)$ Only $(1)$,$(3)$, and $(4)$ are true.
$(C)$ Only $(2)$,$(3)$, and $(4)$ are true.
$(D)$ Only $(2)$,$(3)$, and $(5)$ are true.
$(E)$ Only $(1)$,$(2)$, and $(5)$ are true.
I know that $(1)$ is true, $(2)$ is false and $(3)$ is true. What is the difference between $(4)$ and $(5)$ though? How do I know which one is correct? Apparently statement $(4)$ is the correct one but why is that?
linear-algebra linear-transformations
linear-algebra linear-transformations
edited Dec 23 '18 at 5:05
Future Math person
asked Dec 23 '18 at 4:55
Future Math personFuture Math person
993818
993818
$begingroup$
Hint: think about the rank-nullity theorem.
$endgroup$
– angryavian
Dec 23 '18 at 5:00
$begingroup$
How? I need to know what the rank is and the number of columns to be useful.
$endgroup$
– Future Math person
Dec 23 '18 at 5:02
$begingroup$
Doesn't $mathbb{R}^3 to mathbb{R}^2$ tell you about the shape of the matrix?
$endgroup$
– angryavian
Dec 23 '18 at 5:03
$begingroup$
It does but I'm confused if the number of columns would be 2 or 3. Would it be 2 because that's what I have transformed to?
$endgroup$
– Future Math person
Dec 23 '18 at 5:04
$begingroup$
If $M$ is the matrix and $v in mathbb{R}^3$ then the result of applying $L$ to $v$ is $Mv$.
$endgroup$
– angryavian
Dec 23 '18 at 5:06
add a comment |
$begingroup$
Hint: think about the rank-nullity theorem.
$endgroup$
– angryavian
Dec 23 '18 at 5:00
$begingroup$
How? I need to know what the rank is and the number of columns to be useful.
$endgroup$
– Future Math person
Dec 23 '18 at 5:02
$begingroup$
Doesn't $mathbb{R}^3 to mathbb{R}^2$ tell you about the shape of the matrix?
$endgroup$
– angryavian
Dec 23 '18 at 5:03
$begingroup$
It does but I'm confused if the number of columns would be 2 or 3. Would it be 2 because that's what I have transformed to?
$endgroup$
– Future Math person
Dec 23 '18 at 5:04
$begingroup$
If $M$ is the matrix and $v in mathbb{R}^3$ then the result of applying $L$ to $v$ is $Mv$.
$endgroup$
– angryavian
Dec 23 '18 at 5:06
$begingroup$
Hint: think about the rank-nullity theorem.
$endgroup$
– angryavian
Dec 23 '18 at 5:00
$begingroup$
Hint: think about the rank-nullity theorem.
$endgroup$
– angryavian
Dec 23 '18 at 5:00
$begingroup$
How? I need to know what the rank is and the number of columns to be useful.
$endgroup$
– Future Math person
Dec 23 '18 at 5:02
$begingroup$
How? I need to know what the rank is and the number of columns to be useful.
$endgroup$
– Future Math person
Dec 23 '18 at 5:02
$begingroup$
Doesn't $mathbb{R}^3 to mathbb{R}^2$ tell you about the shape of the matrix?
$endgroup$
– angryavian
Dec 23 '18 at 5:03
$begingroup$
Doesn't $mathbb{R}^3 to mathbb{R}^2$ tell you about the shape of the matrix?
$endgroup$
– angryavian
Dec 23 '18 at 5:03
$begingroup$
It does but I'm confused if the number of columns would be 2 or 3. Would it be 2 because that's what I have transformed to?
$endgroup$
– Future Math person
Dec 23 '18 at 5:04
$begingroup$
It does but I'm confused if the number of columns would be 2 or 3. Would it be 2 because that's what I have transformed to?
$endgroup$
– Future Math person
Dec 23 '18 at 5:04
$begingroup$
If $M$ is the matrix and $v in mathbb{R}^3$ then the result of applying $L$ to $v$ is $Mv$.
$endgroup$
– angryavian
Dec 23 '18 at 5:06
$begingroup$
If $M$ is the matrix and $v in mathbb{R}^3$ then the result of applying $L$ to $v$ is $Mv$.
$endgroup$
– angryavian
Dec 23 '18 at 5:06
add a comment |
2 Answers
2
active
oldest
votes
$begingroup$
Recall that the rank-nullity theorem states that the rank plus the nullity of a linear transformation equals the dimension of its domain.
In terms of the matrix of the linear transformation, the dimension of its columnspace plus the dimension of its nullspace equals the number of columns.
In (4), the matrix is $2 times 3$, so the rank plus nullity equals $3$. The rank must be $le 2$ (do you see why?), so the nullity must be $ge 1$. Thus (4) is true.
In (5), the matrix is $3 times 2$, so the rank plus nullity equals $2$. It is possible for the rank to equal $2$ while the nullity equals $0$; for example consider
$$begin{bmatrix} 1 & 0 \ 0 & 1 \ 0 & 0 end{bmatrix}.$$
So (5) is false.
$endgroup$
$begingroup$
I corrected the question to the proper statement that should be right.
$endgroup$
– Future Math person
Dec 23 '18 at 5:05
$begingroup$
I get everything you said except the size of the matrix. I'm simply not seeing how you know it's $2 times 3$. I feel like it's obvious but I feel I am missing something very obvious.
$endgroup$
– Future Math person
Dec 23 '18 at 5:09
1
$begingroup$
@FutureMathperson a linear transformation from $mathbb{R}^{n}$ to $mathbb{R}^{m}$ is represented by an $mtimes n$ matrix. In this case, $n=3$ and $m=2$
$endgroup$
– pwerth
Dec 23 '18 at 5:15
$begingroup$
I guess I didn't cover that when I took linear algebra. Is there a place I could see the proof of this or an intuitive explanation of why this is the case?
$endgroup$
– Future Math person
Dec 23 '18 at 5:17
1
$begingroup$
I tried to explain in my comment on your question. How does the matrix represent the linear transformation? A linear transformation takes in a vector in one vector space and spits out a vector in another vector space. In terms of the matrix M representing the transformation, it takes in a vector v and spits out Mv. in order for Mv to make sense, the matrix multiplication must be compatible (so the number of columns is the dimension of the domain) and the output must be in the target vector space (so the number of rows must be the dimension of the target space).
$endgroup$
– angryavian
Dec 23 '18 at 5:56
|
show 1 more comment
$begingroup$
As an easy counterexample to $(5)$, take the embedding of $mathbb{R}^{2}$ into $mathbb{R}^{3}$ given by $T(x_{1},x_{2})=(x_{1},x_{2},0)$. This is obviously linear and the null space consists of only the zero vector $(0,0$).
However, if $T:mathbb{R}^{3}tomathbb{R}^{2}$ is linear, the null space must have positive dimension. This follows immediately from the rank-nullity theorem, since the rank of the matrix representing $T$ can be at most $2$, but the rank plus the nullity must equal $3$, the number of columns of this matrix.
Intuitively, if you are going from a bigger space to a smaller one in a linear fashion, a lot of elements are going to have to get killed (mapped to $0$). In particular, the dimension of the elements that are killed (i.e. the nullity of the mapping) has to be at least as big as the difference in dimension between the domain and codomain, which in this case is $3-2=1$.
$endgroup$
$begingroup$
I corrected it. Sorry about that.
$endgroup$
– Future Math person
Dec 23 '18 at 5:06
add a comment |
Your Answer
StackExchange.ready(function() {
var channelOptions = {
tags: "".split(" "),
id: "69"
};
initTagRenderer("".split(" "), "".split(" "), channelOptions);
StackExchange.using("externalEditor", function() {
// Have to fire editor after snippets, if snippets enabled
if (StackExchange.settings.snippets.snippetsEnabled) {
StackExchange.using("snippets", function() {
createEditor();
});
}
else {
createEditor();
}
});
function createEditor() {
StackExchange.prepareEditor({
heartbeatType: 'answer',
autoActivateHeartbeat: false,
convertImagesToLinks: true,
noModals: true,
showLowRepImageUploadWarning: true,
reputationToPostImages: 10,
bindNavPrevention: true,
postfix: "",
imageUploader: {
brandingHtml: "Powered by u003ca class="icon-imgur-white" href="https://imgur.com/"u003eu003c/au003e",
contentPolicyHtml: "User contributions licensed under u003ca href="https://creativecommons.org/licenses/by-sa/3.0/"u003ecc by-sa 3.0 with attribution requiredu003c/au003e u003ca href="https://stackoverflow.com/legal/content-policy"u003e(content policy)u003c/au003e",
allowUrls: true
},
noCode: true, onDemand: true,
discardSelector: ".discard-answer"
,immediatelyShowMarkdownHelp:true
});
}
});
Sign up or log in
StackExchange.ready(function () {
StackExchange.helpers.onClickDraftSave('#login-link');
});
Sign up using Google
Sign up using Facebook
Sign up using Email and Password
Post as a guest
Required, but never shown
StackExchange.ready(
function () {
StackExchange.openid.initPostLogin('.new-post-login', 'https%3a%2f%2fmath.stackexchange.com%2fquestions%2f3050067%2fnullity-of-a-matrix-transformation%23new-answer', 'question_page');
}
);
Post as a guest
Required, but never shown
2 Answers
2
active
oldest
votes
2 Answers
2
active
oldest
votes
active
oldest
votes
active
oldest
votes
$begingroup$
Recall that the rank-nullity theorem states that the rank plus the nullity of a linear transformation equals the dimension of its domain.
In terms of the matrix of the linear transformation, the dimension of its columnspace plus the dimension of its nullspace equals the number of columns.
In (4), the matrix is $2 times 3$, so the rank plus nullity equals $3$. The rank must be $le 2$ (do you see why?), so the nullity must be $ge 1$. Thus (4) is true.
In (5), the matrix is $3 times 2$, so the rank plus nullity equals $2$. It is possible for the rank to equal $2$ while the nullity equals $0$; for example consider
$$begin{bmatrix} 1 & 0 \ 0 & 1 \ 0 & 0 end{bmatrix}.$$
So (5) is false.
$endgroup$
$begingroup$
I corrected the question to the proper statement that should be right.
$endgroup$
– Future Math person
Dec 23 '18 at 5:05
$begingroup$
I get everything you said except the size of the matrix. I'm simply not seeing how you know it's $2 times 3$. I feel like it's obvious but I feel I am missing something very obvious.
$endgroup$
– Future Math person
Dec 23 '18 at 5:09
1
$begingroup$
@FutureMathperson a linear transformation from $mathbb{R}^{n}$ to $mathbb{R}^{m}$ is represented by an $mtimes n$ matrix. In this case, $n=3$ and $m=2$
$endgroup$
– pwerth
Dec 23 '18 at 5:15
$begingroup$
I guess I didn't cover that when I took linear algebra. Is there a place I could see the proof of this or an intuitive explanation of why this is the case?
$endgroup$
– Future Math person
Dec 23 '18 at 5:17
1
$begingroup$
I tried to explain in my comment on your question. How does the matrix represent the linear transformation? A linear transformation takes in a vector in one vector space and spits out a vector in another vector space. In terms of the matrix M representing the transformation, it takes in a vector v and spits out Mv. in order for Mv to make sense, the matrix multiplication must be compatible (so the number of columns is the dimension of the domain) and the output must be in the target vector space (so the number of rows must be the dimension of the target space).
$endgroup$
– angryavian
Dec 23 '18 at 5:56
|
show 1 more comment
$begingroup$
Recall that the rank-nullity theorem states that the rank plus the nullity of a linear transformation equals the dimension of its domain.
In terms of the matrix of the linear transformation, the dimension of its columnspace plus the dimension of its nullspace equals the number of columns.
In (4), the matrix is $2 times 3$, so the rank plus nullity equals $3$. The rank must be $le 2$ (do you see why?), so the nullity must be $ge 1$. Thus (4) is true.
In (5), the matrix is $3 times 2$, so the rank plus nullity equals $2$. It is possible for the rank to equal $2$ while the nullity equals $0$; for example consider
$$begin{bmatrix} 1 & 0 \ 0 & 1 \ 0 & 0 end{bmatrix}.$$
So (5) is false.
$endgroup$
$begingroup$
I corrected the question to the proper statement that should be right.
$endgroup$
– Future Math person
Dec 23 '18 at 5:05
$begingroup$
I get everything you said except the size of the matrix. I'm simply not seeing how you know it's $2 times 3$. I feel like it's obvious but I feel I am missing something very obvious.
$endgroup$
– Future Math person
Dec 23 '18 at 5:09
1
$begingroup$
@FutureMathperson a linear transformation from $mathbb{R}^{n}$ to $mathbb{R}^{m}$ is represented by an $mtimes n$ matrix. In this case, $n=3$ and $m=2$
$endgroup$
– pwerth
Dec 23 '18 at 5:15
$begingroup$
I guess I didn't cover that when I took linear algebra. Is there a place I could see the proof of this or an intuitive explanation of why this is the case?
$endgroup$
– Future Math person
Dec 23 '18 at 5:17
1
$begingroup$
I tried to explain in my comment on your question. How does the matrix represent the linear transformation? A linear transformation takes in a vector in one vector space and spits out a vector in another vector space. In terms of the matrix M representing the transformation, it takes in a vector v and spits out Mv. in order for Mv to make sense, the matrix multiplication must be compatible (so the number of columns is the dimension of the domain) and the output must be in the target vector space (so the number of rows must be the dimension of the target space).
$endgroup$
– angryavian
Dec 23 '18 at 5:56
|
show 1 more comment
$begingroup$
Recall that the rank-nullity theorem states that the rank plus the nullity of a linear transformation equals the dimension of its domain.
In terms of the matrix of the linear transformation, the dimension of its columnspace plus the dimension of its nullspace equals the number of columns.
In (4), the matrix is $2 times 3$, so the rank plus nullity equals $3$. The rank must be $le 2$ (do you see why?), so the nullity must be $ge 1$. Thus (4) is true.
In (5), the matrix is $3 times 2$, so the rank plus nullity equals $2$. It is possible for the rank to equal $2$ while the nullity equals $0$; for example consider
$$begin{bmatrix} 1 & 0 \ 0 & 1 \ 0 & 0 end{bmatrix}.$$
So (5) is false.
$endgroup$
Recall that the rank-nullity theorem states that the rank plus the nullity of a linear transformation equals the dimension of its domain.
In terms of the matrix of the linear transformation, the dimension of its columnspace plus the dimension of its nullspace equals the number of columns.
In (4), the matrix is $2 times 3$, so the rank plus nullity equals $3$. The rank must be $le 2$ (do you see why?), so the nullity must be $ge 1$. Thus (4) is true.
In (5), the matrix is $3 times 2$, so the rank plus nullity equals $2$. It is possible for the rank to equal $2$ while the nullity equals $0$; for example consider
$$begin{bmatrix} 1 & 0 \ 0 & 1 \ 0 & 0 end{bmatrix}.$$
So (5) is false.
answered Dec 23 '18 at 5:04
angryavianangryavian
42.7k23482
42.7k23482
$begingroup$
I corrected the question to the proper statement that should be right.
$endgroup$
– Future Math person
Dec 23 '18 at 5:05
$begingroup$
I get everything you said except the size of the matrix. I'm simply not seeing how you know it's $2 times 3$. I feel like it's obvious but I feel I am missing something very obvious.
$endgroup$
– Future Math person
Dec 23 '18 at 5:09
1
$begingroup$
@FutureMathperson a linear transformation from $mathbb{R}^{n}$ to $mathbb{R}^{m}$ is represented by an $mtimes n$ matrix. In this case, $n=3$ and $m=2$
$endgroup$
– pwerth
Dec 23 '18 at 5:15
$begingroup$
I guess I didn't cover that when I took linear algebra. Is there a place I could see the proof of this or an intuitive explanation of why this is the case?
$endgroup$
– Future Math person
Dec 23 '18 at 5:17
1
$begingroup$
I tried to explain in my comment on your question. How does the matrix represent the linear transformation? A linear transformation takes in a vector in one vector space and spits out a vector in another vector space. In terms of the matrix M representing the transformation, it takes in a vector v and spits out Mv. in order for Mv to make sense, the matrix multiplication must be compatible (so the number of columns is the dimension of the domain) and the output must be in the target vector space (so the number of rows must be the dimension of the target space).
$endgroup$
– angryavian
Dec 23 '18 at 5:56
|
show 1 more comment
$begingroup$
I corrected the question to the proper statement that should be right.
$endgroup$
– Future Math person
Dec 23 '18 at 5:05
$begingroup$
I get everything you said except the size of the matrix. I'm simply not seeing how you know it's $2 times 3$. I feel like it's obvious but I feel I am missing something very obvious.
$endgroup$
– Future Math person
Dec 23 '18 at 5:09
1
$begingroup$
@FutureMathperson a linear transformation from $mathbb{R}^{n}$ to $mathbb{R}^{m}$ is represented by an $mtimes n$ matrix. In this case, $n=3$ and $m=2$
$endgroup$
– pwerth
Dec 23 '18 at 5:15
$begingroup$
I guess I didn't cover that when I took linear algebra. Is there a place I could see the proof of this or an intuitive explanation of why this is the case?
$endgroup$
– Future Math person
Dec 23 '18 at 5:17
1
$begingroup$
I tried to explain in my comment on your question. How does the matrix represent the linear transformation? A linear transformation takes in a vector in one vector space and spits out a vector in another vector space. In terms of the matrix M representing the transformation, it takes in a vector v and spits out Mv. in order for Mv to make sense, the matrix multiplication must be compatible (so the number of columns is the dimension of the domain) and the output must be in the target vector space (so the number of rows must be the dimension of the target space).
$endgroup$
– angryavian
Dec 23 '18 at 5:56
$begingroup$
I corrected the question to the proper statement that should be right.
$endgroup$
– Future Math person
Dec 23 '18 at 5:05
$begingroup$
I corrected the question to the proper statement that should be right.
$endgroup$
– Future Math person
Dec 23 '18 at 5:05
$begingroup$
I get everything you said except the size of the matrix. I'm simply not seeing how you know it's $2 times 3$. I feel like it's obvious but I feel I am missing something very obvious.
$endgroup$
– Future Math person
Dec 23 '18 at 5:09
$begingroup$
I get everything you said except the size of the matrix. I'm simply not seeing how you know it's $2 times 3$. I feel like it's obvious but I feel I am missing something very obvious.
$endgroup$
– Future Math person
Dec 23 '18 at 5:09
1
1
$begingroup$
@FutureMathperson a linear transformation from $mathbb{R}^{n}$ to $mathbb{R}^{m}$ is represented by an $mtimes n$ matrix. In this case, $n=3$ and $m=2$
$endgroup$
– pwerth
Dec 23 '18 at 5:15
$begingroup$
@FutureMathperson a linear transformation from $mathbb{R}^{n}$ to $mathbb{R}^{m}$ is represented by an $mtimes n$ matrix. In this case, $n=3$ and $m=2$
$endgroup$
– pwerth
Dec 23 '18 at 5:15
$begingroup$
I guess I didn't cover that when I took linear algebra. Is there a place I could see the proof of this or an intuitive explanation of why this is the case?
$endgroup$
– Future Math person
Dec 23 '18 at 5:17
$begingroup$
I guess I didn't cover that when I took linear algebra. Is there a place I could see the proof of this or an intuitive explanation of why this is the case?
$endgroup$
– Future Math person
Dec 23 '18 at 5:17
1
1
$begingroup$
I tried to explain in my comment on your question. How does the matrix represent the linear transformation? A linear transformation takes in a vector in one vector space and spits out a vector in another vector space. In terms of the matrix M representing the transformation, it takes in a vector v and spits out Mv. in order for Mv to make sense, the matrix multiplication must be compatible (so the number of columns is the dimension of the domain) and the output must be in the target vector space (so the number of rows must be the dimension of the target space).
$endgroup$
– angryavian
Dec 23 '18 at 5:56
$begingroup$
I tried to explain in my comment on your question. How does the matrix represent the linear transformation? A linear transformation takes in a vector in one vector space and spits out a vector in another vector space. In terms of the matrix M representing the transformation, it takes in a vector v and spits out Mv. in order for Mv to make sense, the matrix multiplication must be compatible (so the number of columns is the dimension of the domain) and the output must be in the target vector space (so the number of rows must be the dimension of the target space).
$endgroup$
– angryavian
Dec 23 '18 at 5:56
|
show 1 more comment
$begingroup$
As an easy counterexample to $(5)$, take the embedding of $mathbb{R}^{2}$ into $mathbb{R}^{3}$ given by $T(x_{1},x_{2})=(x_{1},x_{2},0)$. This is obviously linear and the null space consists of only the zero vector $(0,0$).
However, if $T:mathbb{R}^{3}tomathbb{R}^{2}$ is linear, the null space must have positive dimension. This follows immediately from the rank-nullity theorem, since the rank of the matrix representing $T$ can be at most $2$, but the rank plus the nullity must equal $3$, the number of columns of this matrix.
Intuitively, if you are going from a bigger space to a smaller one in a linear fashion, a lot of elements are going to have to get killed (mapped to $0$). In particular, the dimension of the elements that are killed (i.e. the nullity of the mapping) has to be at least as big as the difference in dimension between the domain and codomain, which in this case is $3-2=1$.
$endgroup$
$begingroup$
I corrected it. Sorry about that.
$endgroup$
– Future Math person
Dec 23 '18 at 5:06
add a comment |
$begingroup$
As an easy counterexample to $(5)$, take the embedding of $mathbb{R}^{2}$ into $mathbb{R}^{3}$ given by $T(x_{1},x_{2})=(x_{1},x_{2},0)$. This is obviously linear and the null space consists of only the zero vector $(0,0$).
However, if $T:mathbb{R}^{3}tomathbb{R}^{2}$ is linear, the null space must have positive dimension. This follows immediately from the rank-nullity theorem, since the rank of the matrix representing $T$ can be at most $2$, but the rank plus the nullity must equal $3$, the number of columns of this matrix.
Intuitively, if you are going from a bigger space to a smaller one in a linear fashion, a lot of elements are going to have to get killed (mapped to $0$). In particular, the dimension of the elements that are killed (i.e. the nullity of the mapping) has to be at least as big as the difference in dimension between the domain and codomain, which in this case is $3-2=1$.
$endgroup$
$begingroup$
I corrected it. Sorry about that.
$endgroup$
– Future Math person
Dec 23 '18 at 5:06
add a comment |
$begingroup$
As an easy counterexample to $(5)$, take the embedding of $mathbb{R}^{2}$ into $mathbb{R}^{3}$ given by $T(x_{1},x_{2})=(x_{1},x_{2},0)$. This is obviously linear and the null space consists of only the zero vector $(0,0$).
However, if $T:mathbb{R}^{3}tomathbb{R}^{2}$ is linear, the null space must have positive dimension. This follows immediately from the rank-nullity theorem, since the rank of the matrix representing $T$ can be at most $2$, but the rank plus the nullity must equal $3$, the number of columns of this matrix.
Intuitively, if you are going from a bigger space to a smaller one in a linear fashion, a lot of elements are going to have to get killed (mapped to $0$). In particular, the dimension of the elements that are killed (i.e. the nullity of the mapping) has to be at least as big as the difference in dimension between the domain and codomain, which in this case is $3-2=1$.
$endgroup$
As an easy counterexample to $(5)$, take the embedding of $mathbb{R}^{2}$ into $mathbb{R}^{3}$ given by $T(x_{1},x_{2})=(x_{1},x_{2},0)$. This is obviously linear and the null space consists of only the zero vector $(0,0$).
However, if $T:mathbb{R}^{3}tomathbb{R}^{2}$ is linear, the null space must have positive dimension. This follows immediately from the rank-nullity theorem, since the rank of the matrix representing $T$ can be at most $2$, but the rank plus the nullity must equal $3$, the number of columns of this matrix.
Intuitively, if you are going from a bigger space to a smaller one in a linear fashion, a lot of elements are going to have to get killed (mapped to $0$). In particular, the dimension of the elements that are killed (i.e. the nullity of the mapping) has to be at least as big as the difference in dimension between the domain and codomain, which in this case is $3-2=1$.
edited Dec 23 '18 at 5:06
answered Dec 23 '18 at 5:05
pwerthpwerth
3,340417
3,340417
$begingroup$
I corrected it. Sorry about that.
$endgroup$
– Future Math person
Dec 23 '18 at 5:06
add a comment |
$begingroup$
I corrected it. Sorry about that.
$endgroup$
– Future Math person
Dec 23 '18 at 5:06
$begingroup$
I corrected it. Sorry about that.
$endgroup$
– Future Math person
Dec 23 '18 at 5:06
$begingroup$
I corrected it. Sorry about that.
$endgroup$
– Future Math person
Dec 23 '18 at 5:06
add a comment |
Thanks for contributing an answer to Mathematics Stack Exchange!
- Please be sure to answer the question. Provide details and share your research!
But avoid …
- Asking for help, clarification, or responding to other answers.
- Making statements based on opinion; back them up with references or personal experience.
Use MathJax to format equations. MathJax reference.
To learn more, see our tips on writing great answers.
Sign up or log in
StackExchange.ready(function () {
StackExchange.helpers.onClickDraftSave('#login-link');
});
Sign up using Google
Sign up using Facebook
Sign up using Email and Password
Post as a guest
Required, but never shown
StackExchange.ready(
function () {
StackExchange.openid.initPostLogin('.new-post-login', 'https%3a%2f%2fmath.stackexchange.com%2fquestions%2f3050067%2fnullity-of-a-matrix-transformation%23new-answer', 'question_page');
}
);
Post as a guest
Required, but never shown
Sign up or log in
StackExchange.ready(function () {
StackExchange.helpers.onClickDraftSave('#login-link');
});
Sign up using Google
Sign up using Facebook
Sign up using Email and Password
Post as a guest
Required, but never shown
Sign up or log in
StackExchange.ready(function () {
StackExchange.helpers.onClickDraftSave('#login-link');
});
Sign up using Google
Sign up using Facebook
Sign up using Email and Password
Post as a guest
Required, but never shown
Sign up or log in
StackExchange.ready(function () {
StackExchange.helpers.onClickDraftSave('#login-link');
});
Sign up using Google
Sign up using Facebook
Sign up using Email and Password
Sign up using Google
Sign up using Facebook
Sign up using Email and Password
Post as a guest
Required, but never shown
Required, but never shown
Required, but never shown
Required, but never shown
Required, but never shown
Required, but never shown
Required, but never shown
Required, but never shown
Required, but never shown
k v,HuDx8PVzOWXNozKsfF8,R9tgmYk8G8,TF3,ipup
$begingroup$
Hint: think about the rank-nullity theorem.
$endgroup$
– angryavian
Dec 23 '18 at 5:00
$begingroup$
How? I need to know what the rank is and the number of columns to be useful.
$endgroup$
– Future Math person
Dec 23 '18 at 5:02
$begingroup$
Doesn't $mathbb{R}^3 to mathbb{R}^2$ tell you about the shape of the matrix?
$endgroup$
– angryavian
Dec 23 '18 at 5:03
$begingroup$
It does but I'm confused if the number of columns would be 2 or 3. Would it be 2 because that's what I have transformed to?
$endgroup$
– Future Math person
Dec 23 '18 at 5:04
$begingroup$
If $M$ is the matrix and $v in mathbb{R}^3$ then the result of applying $L$ to $v$ is $Mv$.
$endgroup$
– angryavian
Dec 23 '18 at 5:06